parent
d8718370e9
commit
f64ea53319
@ -1,53 +1,163 @@
|
|||||||
# Introduction to Time Series Forecasting
|
# Introduction to Time Series Forecasting
|
||||||
|
|
||||||

|
|
||||||
[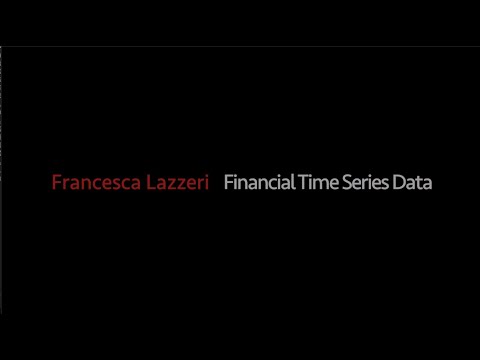](https://youtu.be/mAv1SEXUKhE "Introduction to Time Series Forecasting")
|
[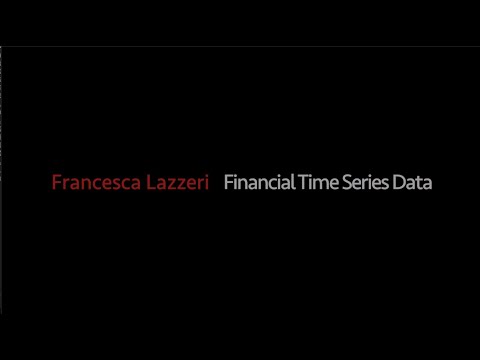](https://youtu.be/mAv1SEXUKhE "Introduction to Time Series Forecasting")
|
||||||
|
|
||||||
> Introduction to Time Series Forecasting with Francesca Lazzeri; starting at 7:13.
|
> Introduction to Time Series Forecasting with Francesca Lazzeri; starting at 7:13.
|
||||||
|
|
||||||
## [Pre-lecture quiz](link-to-quiz-app)
|
## [Pre-lecture quiz](link-to-quiz-app)
|
||||||
|
|
||||||
In this lesson and the following one, you will learn a bit about Time Series Forecasting, an interesting and valuable part of a ML scientist's repertoire that is a bit lesser known than other topics. Time Series Forecasting is a sort of crystal ball: based on past performance of a variable such as price, you can predict its future potential value. It's a powerful and interesting field especially in business, unsurprisingly, given its direct application to problems of value. While deep learning techniques have started to be used to gain more insights in the prediction of future performance, it remains a field greatly informed by classic ML techniques.
|
In this lesson and the following one, you will learn a bit about Time Series Forecasting, an interesting and valuable part of a ML scientist's repertoire that is a bit lesser known than other topics. Time Series Forecasting is a sort of crystal ball: based on past performance of a variable such as price, you can predict its future potential value.
|
||||||
|
|
||||||
|
It's a powerful and interesting field especially in business, given its direct application to problems of value, pricing, inventory, and supply chain issues. While deep learning techniques have started to be used to gain more insights in the prediction of future performance, Time Series Forecasting remains a field greatly informed by classic ML techniques.
|
||||||
|
|
||||||
|
> Penn State's useful Time Series curriculum can be found [here](https://online.stat.psu.edu/stat510/lesson/1)
|
||||||
### Introduction
|
### Introduction
|
||||||
|
|
||||||
Supposing you maintain an array of smart parking meters that provide data about how often they are used and for how long over time. What if you could generate revenue to maintain your streets by tweaking the prices of the meters when there is greater demand for them? What if you could predict, based on the meter's past performance, its future value according to the laws of supply and demand? This is a challenge that could be tackled by a Time Series problem. It wouldn't make those in search of a rare parking spot in busy times very happy to have to pay more for it, but it would be a sure way to generate revenue to clean the streets!
|
Supposing you maintain an array of smart parking meters that provide data about how often they are used and for how long over time. What if you could generate revenue to maintain your streets by slightly augmenting the prices of the meters when there is greater demand for them? What if you could predict, based on the meter's past performance, its future value according to the laws of supply and demand? This is a challenge that could be tackled by Time Series Forecasting. It wouldn't make those folks in search of a rare parking spot in busy times very happy to have to pay more for it, but it would be a sure way to generate revenue to clean the streets!
|
||||||
|
|
||||||
Let's explore some of the types of Time Series algorithms and start a notebook in preparation of cleaning some data. The data you will analyze is taken from the GEFCom2014 forecasting competition. It consists of 3 years of hourly electricity load and temperature values between 2012 and 2014. Given the historical patterns of electricity load and temperature, you can predict future values of electricity load. In this example, you'll learn how to forecast one time step ahead, using historical load data only.
|
Let's explore some of the types of Time Series algorithms and start a notebook to clean and prepare some data. The data you will analyze is taken from the GEFCom2014 forecasting competition. It consists of 3 years of hourly electricity load and temperature values between 2012 and 2014. Given the historical patterns of electricity load and temperature, you can predict future values of electricity load. In this example, you'll learn how to forecast one time step ahead, using historical load data only.
|
||||||
|
|
||||||
Before starting, however, it's useful to understand what's going on behind the scenes.
|
Before starting, however, it's useful to understand what's going on behind the scenes.
|
||||||
|
|
||||||
## Types of Time Series Forecasting
|
## Some Definitions
|
||||||
|
|
||||||
|
When encountering the term 'time series' you need to understand its use in several different contexts.
|
||||||
|
### Time Series
|
||||||
|
|
||||||
|
In mathematics, "a time series is a series of data points indexed (or listed or graphed) in time order. Most commonly, a time series is a sequence taken at successive equally spaced points in time." An example of a time series is the daily closing value of the Dow Jones Industrial Average.[source](https://en.wikipedia.org/wiki/Time_series). The use of time series plots and statistical modeling is frequently encountered in signal processing, weather forecasting, earthquake prediction, and other fields where events occur and data points can be plotted over time.
|
||||||
|
### Time Series Analysis
|
||||||
|
|
||||||
|
Time Series Analysis is the analysis of the above mentioned time series data. Time series data can take distinct forms, including 'interrupted time series' which detects patterns in a time series' evolution before and after an interrupting event. The type of analysis needed for the time series depends on the nature of the data. Time series data itself can take the form of series of numbers or characters.
|
||||||
|
|
||||||
|
The analysis be performed using a variety of methods, including frequency-domain and time-domain, linear and nonlinear, and more. [Learn more](https://www.itl.nist.gov/div898/handbook/pmc/section4/pmc4.htm) about the may ways to analyze this type of data.
|
||||||
|
### Time Series Forecasting
|
||||||
|
|
||||||
|
Time Series Forecasting is the use of a model to predict future values based on patterns displayed by previously gathered data as it occurred in the past. While it is possible to use regression models to explore time series data, with time indices as x variables on a plot, this type of data is best analyzed using special types of models.
|
||||||
|
|
||||||
|
Time series data is a list of ordered observations, unlike data that can be analyzed by linear regression. The most common one is ARIMA, an acronym that stands for "Autoregressive Integrated Moving Average".
|
||||||
|
ARIMA models "relate the present value of a series to past values and past prediction errors." [source](https://online.stat.psu.edu/stat510/lesson/1/1.1). They are most appropriate for analyzing time-domain data, where data is ordered over time.
|
||||||
|
|
||||||
|
> There are several types of ARIMA models, which you can learn about [here](https://people.duke.edu/~rnau/411arim.htm) and which you will touch on in the next lesson.
|
||||||
|
|
||||||
|
In the next lesson, you will build an ARIMA model using [Univariate Time Series](https://itl.nist.gov/div898/handbook/pmc/section4/pmc44.htm), which focuses on one variable that changes its value over time. An example of this type of data is [this dataset](https://itl.nist.gov/div898/handbook/pmc/section4/pmc4411.htm) that records the monthly C02 concentration at the Mauna Loa Observatory:
|
||||||
|
|
||||||
|
| CO2 | YearMonth | Year | Month |
|
||||||
|
| :----: | :-------: | :---: | :---: |
|
||||||
|
| 330.62 | 1975.04 | 1975 | 1 |
|
||||||
|
| 331.40 | 1975.13 | 1975 | 2 |
|
||||||
|
| 331.87 | 1975.21 | 1975 | 3 |
|
||||||
|
| 333.18 | 1975.29 | 1975 | 4 |
|
||||||
|
| 333.92 | 1975.38 | 1975 | 5 |
|
||||||
|
| 333.43 | 1975.46 | 1975 | 6 |
|
||||||
|
| 331.85 | 1975.54 | 1975 | 7 |
|
||||||
|
| 330.01 | 1975.63 | 1975 | 8 |
|
||||||
|
| 328.51 | 1975.71 | 1975 | 9 |
|
||||||
|
| 328.41 | 1975.79 | 1975 | 10 |
|
||||||
|
| 329.25 | 1975.88 | 1975 | 11 |
|
||||||
|
| 330.97 | 1975.96 | 1975 | 12 |
|
||||||
|
|
||||||
|
✅ Identify the variable that changes over time in this dataset
|
||||||
|
|
||||||
|
|
||||||
|
## Time Series [data characteristics](https://online.stat.psu.edu/stat510/lesson/1/1.1) to consider
|
||||||
|
|
||||||
|
When looking at time series data, you might notice that it has certain characteristics that you need to take into account and mitigate to better understand its patterns. If you consider time series data as potentially providing a 'signal' that you want to analyze, these characteristics can be thought of as 'noise'. You often will need to reduce this 'noise' by offsetting some of these characteristics using some statistical techniques.
|
||||||
|
### Trends
|
||||||
|
Measurable increases and decreases over time
|
||||||
|
### [Seasonality](https://machinelearningmastery.com/time-series-seasonality-with-python/)
|
||||||
|
Periodic fluctuations, such as holiday rushes that might affect sales, for example. [Take a look](https://itl.nist.gov/div898/handbook/pmc/section4/pmc443.htm) at how different types of plots display seasonality in data.
|
||||||
|
### Outliers
|
||||||
|
Outliers are far away from the standard data variance.
|
||||||
|
### Long-run cycle
|
||||||
|
Independent of seasonality, data might display a long-run cycle such as an economic down-turn that lasts longer than a year.
|
||||||
|
### Constant variance
|
||||||
|
Over time, some data display constant fluctuations, such as energy usage per day and night.
|
||||||
|
### Abrupt changes
|
||||||
|
The data might display an abrupt change that might need further analysis. The abrupt shuttering of businesses due to COVID, for example, caused changes in data.
|
||||||
|
|
||||||
## Algorithms
|
### Stationary
|
||||||
|
|
||||||
## Some Math
|
✅ Here is a [sample time series plot](https://www.kaggle.com/kashnitsky/topic-9-part-1-time-series-analysis-in-python) showing daily in-game currency spent over a few years. Can you identify any of the characteristics listed above in this data?
|
||||||
|
|
||||||
## ARIMA
|

|
||||||
|
|
||||||
## [Topic 1]
|
## Getting started with power usage data
|
||||||
|
|
||||||
### Task:
|
Let's get started creating a time series model to predict future power usage given past usage.
|
||||||
|
|
||||||
Work together to progressively enhance your codebase to build the project with shared code:
|
> The data in this example is taken from the GEFCom2014 forecasting competition. It consists of 3 years of hourly electricity load and temperature values between 2012 and 2014.
|
||||||
|
>
|
||||||
|
> Tao Hong, Pierre Pinson, Shu Fan, Hamidreza Zareipour, Alberto Troccoli and Rob J. Hyndman, "Probabilistic energy forecasting: Global Energy Forecasting Competition 2014 and beyond", International Journal of Forecasting, vol.32, no.3, pp 896-913, July-September, 2016.
|
||||||
|
|
||||||
```html
|
In the `working` folder of this lesson, open the `notebook.ipynb` file. Start by adding libraries that will help you load and visualize remote data
|
||||||
code blocks
|
|
||||||
|
```python
|
||||||
|
import os
|
||||||
|
import matplotlib.pyplot as plt
|
||||||
|
from common.utils import load_data
|
||||||
|
from common.extract_data import extract_data
|
||||||
|
%matplotlib inline
|
||||||
```
|
```
|
||||||
|
Note, you are using the files from the included `common` folder which set up your environment and handle downloading the data.
|
||||||
|
|
||||||
✅ Knowledge Check - use this moment to stretch students' knowledge with open questions
|
Next, download, move and extract the zip file of data:
|
||||||
|
|
||||||
## [Topic 2]
|
```python
|
||||||
|
data_dir = './data'
|
||||||
|
|
||||||
## [Topic 3]
|
if not os.path.exists(os.path.join(data_dir, 'energy.csv')):
|
||||||
|
# Download and move the zip file
|
||||||
|
!wget https://www.dropbox.com/s/pqenrr2mcvl0hk9/GEFCom2014.zip
|
||||||
|
!mv GEFCom2014.zip ./data
|
||||||
|
# If not done already, extract zipped data and save as csv
|
||||||
|
extract_data(data_dir)
|
||||||
|
```
|
||||||
|
Take a look at the way the data looks:
|
||||||
|
|
||||||
## 🚀Challenge
|
```
|
||||||
|
energy = load_data(data_dir)[['load']]
|
||||||
|
energy.head()
|
||||||
|
```
|
||||||
|
You can see that there are two columns representing date and load:
|
||||||
|
|
||||||
|
| date | load |
|
||||||
|
| :-----------------: | :----: |
|
||||||
|
| 2012-01-01 00:00:00 | 2698.0 |
|
||||||
|
| 2012-01-01 01:00:00 | 2558.0 |
|
||||||
|
| 2012-01-01 02:00:00 | 2444.0 |
|
||||||
|
| 2012-01-01 03:00:00 | 2402.0 |
|
||||||
|
| 2012-01-01 04:00:00 | 2403.0 |
|
||||||
|
|
||||||
|
Now, plot the data:
|
||||||
|
|
||||||
|
```python
|
||||||
|
energy.plot(y='load', subplots=True, figsize=(15, 8), fontsize=12)
|
||||||
|
plt.xlabel('timestamp', fontsize=12)
|
||||||
|
plt.ylabel('load', fontsize=12)
|
||||||
|
plt.show()
|
||||||
|
```
|
||||||
|

|
||||||
|
|
||||||
|
Now, plot the first week of July 2014
|
||||||
|
|
||||||
Add a challenge for students to work on collaboratively in class to enhance the project
|
```python
|
||||||
|
energy['2014-07-01':'2014-07-07'].plot(y='load', subplots=True, figsize=(15, 8), fontsize=12)
|
||||||
|
plt.xlabel('timestamp', fontsize=12)
|
||||||
|
plt.ylabel('load', fontsize=12)
|
||||||
|
plt.show()
|
||||||
|
```
|
||||||
|
|
||||||
Optional: add a screenshot of the completed lesson's UI if appropriate
|

|
||||||
|
|
||||||
## [Post-lecture quiz](link-to-quiz-app)
|
A beautiful plot! Take a look at these plots and see if you can determine any of the characteristics listed above. What can we surmise just by visualizing the data?
|
||||||
|
|
||||||
|
In the next lesson, you will create an ARIMA model to create some forecasts.
|
||||||
|
|
||||||
|
## 🚀Challenge
|
||||||
|
|
||||||
|
tbd
|
||||||
|
|
||||||
|
## [Post-lecture quiz](link-to-quiz-app)
|
||||||
## Review & Self Study
|
## Review & Self Study
|
||||||
|
|
||||||
**Assignment**: [Assignment Name](assignment.md)
|
**Assignment**: [Assignment Name](assignment.md)
|
||||||
|
After Width: | Height: | Size: 71 KiB |
After Width: | Height: | Size: 45 KiB |
After Width: | Height: | Size: 43 KiB |
Binary file not shown.
Binary file not shown.
Binary file not shown.
@ -0,0 +1,28 @@
|
|||||||
|
# To create the conda environment:
|
||||||
|
# $ conda env create -f environment.yaml
|
||||||
|
#
|
||||||
|
# To update the conda environment:
|
||||||
|
# $ conda env update -f environment.yaml
|
||||||
|
#
|
||||||
|
# To register the conda environment in Jupyter:
|
||||||
|
# $ conda activate dlts
|
||||||
|
# $ python -m ipykernel install --user --name dlts --display-name "Python (dlts)"
|
||||||
|
|
||||||
|
name: dlts
|
||||||
|
channels:
|
||||||
|
- defaults
|
||||||
|
dependencies:
|
||||||
|
- python==3.6.6
|
||||||
|
- pip>=19.1.1
|
||||||
|
- ipykernel>=4.6.1
|
||||||
|
- jupyter>=1.0.0
|
||||||
|
- matplotlib==3.0.0
|
||||||
|
- numpy==1.16.2
|
||||||
|
- pandas==0.23.4
|
||||||
|
- tensorflow==1.12.0
|
||||||
|
- keras==2.2.4
|
||||||
|
- scikit-learn==0.20.3
|
||||||
|
- statsmodels==0.9.0
|
||||||
|
- xlrd >= 1.0.0
|
||||||
|
- pip:
|
||||||
|
- pyramid-arima==0.8.1
|
@ -0,0 +1,37 @@
|
|||||||
|
import zipfile
|
||||||
|
import os
|
||||||
|
import sys
|
||||||
|
import pandas as pd
|
||||||
|
|
||||||
|
# This function unzips the GEFCom2014 data zip file and extracts the 'extended'
|
||||||
|
# load forecasting competition data. Data is saved in energy.csv
|
||||||
|
def extract_data(data_dir):
|
||||||
|
GEFCom_dir = os.path.join(data_dir, 'GEFCom2014', 'GEFCom2014 Data')
|
||||||
|
|
||||||
|
GEFCom_zipfile = os.path.join(data_dir, 'GEFCom2014.zip')
|
||||||
|
if not os.path.exists(GEFCom_zipfile):
|
||||||
|
sys.exit("Download GEFCom2014.zip from https://www.dropbox.com/s/pqenrr2mcvl0hk9/GEFCom2014.zip?dl=0 and save it to the '{}' directory.".format(data_dir))
|
||||||
|
|
||||||
|
# unzip root directory
|
||||||
|
zip_ref = zipfile.ZipFile(GEFCom_zipfile, 'r')
|
||||||
|
zip_ref.extractall(os.path.join(data_dir, 'GEFCom2014'))
|
||||||
|
zip_ref.close()
|
||||||
|
|
||||||
|
# extract the extended competition data
|
||||||
|
zip_ref = zipfile.ZipFile(os.path.join(GEFCom_dir, 'GEFCom2014-E_V2.zip'), 'r')
|
||||||
|
zip_ref.extractall(os.path.join(data_dir, 'GEFCom2014-E'))
|
||||||
|
zip_ref.close()
|
||||||
|
|
||||||
|
# load the data from Excel file
|
||||||
|
data = pd.read_excel(os.path.join(data_dir, 'GEFCom2014-E', 'GEFCom2014-E.xlsx'), parse_date='Date')
|
||||||
|
|
||||||
|
# create timestamp variable from Date and Hour
|
||||||
|
data['timestamp'] = data['Date'].add(pd.to_timedelta(data.Hour - 1, unit='h'))
|
||||||
|
data = data[['timestamp', 'load', 'T']]
|
||||||
|
data = data.rename(columns={'T':'temp'})
|
||||||
|
|
||||||
|
# remove time period with no load data
|
||||||
|
data = data[data.timestamp >= '2012-01-01']
|
||||||
|
|
||||||
|
# save to csv
|
||||||
|
data.to_csv(os.path.join(data_dir, 'energy.csv'), index=False)
|
@ -0,0 +1,145 @@
|
|||||||
|
import numpy as np
|
||||||
|
import pandas as pd
|
||||||
|
import os
|
||||||
|
from collections import UserDict
|
||||||
|
|
||||||
|
def load_data(data_dir):
|
||||||
|
"""Load the GEFCom 2014 energy load data"""
|
||||||
|
|
||||||
|
energy = pd.read_csv(os.path.join(data_dir, 'energy.csv'), parse_dates=['timestamp'])
|
||||||
|
|
||||||
|
# Reindex the dataframe such that the dataframe has a record for every time point
|
||||||
|
# between the minimum and maximum timestamp in the time series. This helps to
|
||||||
|
# identify missing time periods in the data (there are none in this dataset).
|
||||||
|
|
||||||
|
energy.index = energy['timestamp']
|
||||||
|
energy = energy.reindex(pd.date_range(min(energy['timestamp']),
|
||||||
|
max(energy['timestamp']),
|
||||||
|
freq='H'))
|
||||||
|
energy = energy.drop('timestamp', axis=1)
|
||||||
|
|
||||||
|
return energy
|
||||||
|
|
||||||
|
|
||||||
|
def mape(predictions, actuals):
|
||||||
|
"""Mean absolute percentage error"""
|
||||||
|
return ((predictions - actuals).abs() / actuals).mean()
|
||||||
|
|
||||||
|
|
||||||
|
def create_evaluation_df(predictions, test_inputs, H, scaler):
|
||||||
|
"""Create a data frame for easy evaluation"""
|
||||||
|
eval_df = pd.DataFrame(predictions, columns=['t+'+str(t) for t in range(1, H+1)])
|
||||||
|
eval_df['timestamp'] = test_inputs.dataframe.index
|
||||||
|
eval_df = pd.melt(eval_df, id_vars='timestamp', value_name='prediction', var_name='h')
|
||||||
|
eval_df['actual'] = np.transpose(test_inputs['target']).ravel()
|
||||||
|
eval_df[['prediction', 'actual']] = scaler.inverse_transform(eval_df[['prediction', 'actual']])
|
||||||
|
return eval_df
|
||||||
|
|
||||||
|
|
||||||
|
class TimeSeriesTensor(UserDict):
|
||||||
|
"""A dictionary of tensors for input into the RNN model.
|
||||||
|
|
||||||
|
Use this class to:
|
||||||
|
1. Shift the values of the time series to create a Pandas dataframe containing all the data
|
||||||
|
for a single training example
|
||||||
|
2. Discard any samples with missing values
|
||||||
|
3. Transform this Pandas dataframe into a numpy array of shape
|
||||||
|
(samples, time steps, features) for input into Keras
|
||||||
|
|
||||||
|
The class takes the following parameters:
|
||||||
|
- **dataset**: original time series
|
||||||
|
- **target** name of the target column
|
||||||
|
- **H**: the forecast horizon
|
||||||
|
- **tensor_structures**: a dictionary discribing the tensor structure of the form
|
||||||
|
{ 'tensor_name' : (range(max_backward_shift, max_forward_shift), [feature, feature, ...] ) }
|
||||||
|
if features are non-sequential and should not be shifted, use the form
|
||||||
|
{ 'tensor_name' : (None, [feature, feature, ...])}
|
||||||
|
- **freq**: time series frequency (default 'H' - hourly)
|
||||||
|
- **drop_incomplete**: (Boolean) whether to drop incomplete samples (default True)
|
||||||
|
"""
|
||||||
|
|
||||||
|
def __init__(self, dataset, target, H, tensor_structure, freq='H', drop_incomplete=True):
|
||||||
|
self.dataset = dataset
|
||||||
|
self.target = target
|
||||||
|
self.tensor_structure = tensor_structure
|
||||||
|
self.tensor_names = list(tensor_structure.keys())
|
||||||
|
|
||||||
|
self.dataframe = self._shift_data(H, freq, drop_incomplete)
|
||||||
|
self.data = self._df2tensors(self.dataframe)
|
||||||
|
|
||||||
|
def _shift_data(self, H, freq, drop_incomplete):
|
||||||
|
|
||||||
|
# Use the tensor_structures definitions to shift the features in the original dataset.
|
||||||
|
# The result is a Pandas dataframe with multi-index columns in the hierarchy
|
||||||
|
# tensor - the name of the input tensor
|
||||||
|
# feature - the input feature to be shifted
|
||||||
|
# time step - the time step for the RNN in which the data is input. These labels
|
||||||
|
# are centred on time t. the forecast creation time
|
||||||
|
df = self.dataset.copy()
|
||||||
|
|
||||||
|
idx_tuples = []
|
||||||
|
for t in range(1, H+1):
|
||||||
|
df['t+'+str(t)] = df[self.target].shift(t*-1, freq=freq)
|
||||||
|
idx_tuples.append(('target', 'y', 't+'+str(t)))
|
||||||
|
|
||||||
|
for name, structure in self.tensor_structure.items():
|
||||||
|
rng = structure[0]
|
||||||
|
dataset_cols = structure[1]
|
||||||
|
|
||||||
|
for col in dataset_cols:
|
||||||
|
|
||||||
|
# do not shift non-sequential 'static' features
|
||||||
|
if rng is None:
|
||||||
|
df['context_'+col] = df[col]
|
||||||
|
idx_tuples.append((name, col, 'static'))
|
||||||
|
|
||||||
|
else:
|
||||||
|
for t in rng:
|
||||||
|
sign = '+' if t > 0 else ''
|
||||||
|
shift = str(t) if t != 0 else ''
|
||||||
|
period = 't'+sign+shift
|
||||||
|
shifted_col = name+'_'+col+'_'+period
|
||||||
|
df[shifted_col] = df[col].shift(t*-1, freq=freq)
|
||||||
|
idx_tuples.append((name, col, period))
|
||||||
|
|
||||||
|
df = df.drop(self.dataset.columns, axis=1)
|
||||||
|
idx = pd.MultiIndex.from_tuples(idx_tuples, names=['tensor', 'feature', 'time step'])
|
||||||
|
df.columns = idx
|
||||||
|
|
||||||
|
if drop_incomplete:
|
||||||
|
df = df.dropna(how='any')
|
||||||
|
|
||||||
|
return df
|
||||||
|
|
||||||
|
def _df2tensors(self, dataframe):
|
||||||
|
|
||||||
|
# Transform the shifted Pandas dataframe into the multidimensional numpy arrays. These
|
||||||
|
# arrays can be used to input into the keras model and can be accessed by tensor name.
|
||||||
|
# For example, for a TimeSeriesTensor object named "model_inputs" and a tensor named
|
||||||
|
# "target", the input tensor can be acccessed with model_inputs['target']
|
||||||
|
|
||||||
|
inputs = {}
|
||||||
|
y = dataframe['target']
|
||||||
|
y = y.as_matrix()
|
||||||
|
inputs['target'] = y
|
||||||
|
|
||||||
|
for name, structure in self.tensor_structure.items():
|
||||||
|
rng = structure[0]
|
||||||
|
cols = structure[1]
|
||||||
|
tensor = dataframe[name][cols].as_matrix()
|
||||||
|
if rng is None:
|
||||||
|
tensor = tensor.reshape(tensor.shape[0], len(cols))
|
||||||
|
else:
|
||||||
|
tensor = tensor.reshape(tensor.shape[0], len(cols), len(rng))
|
||||||
|
tensor = np.transpose(tensor, axes=[0, 2, 1])
|
||||||
|
inputs[name] = tensor
|
||||||
|
|
||||||
|
return inputs
|
||||||
|
|
||||||
|
def subset_data(self, new_dataframe):
|
||||||
|
|
||||||
|
# Use this function to recreate the input tensors if the shifted dataframe
|
||||||
|
# has been filtered.
|
||||||
|
|
||||||
|
self.dataframe = new_dataframe
|
||||||
|
self.data = self._df2tensors(self.dataframe)
|
Binary file not shown.
Binary file not shown.
Binary file not shown.
@ -0,0 +1,28 @@
|
|||||||
|
# To create the conda environment:
|
||||||
|
# $ conda env create -f environment.yaml
|
||||||
|
#
|
||||||
|
# To update the conda environment:
|
||||||
|
# $ conda env update -f environment.yaml
|
||||||
|
#
|
||||||
|
# To register the conda environment in Jupyter:
|
||||||
|
# $ conda activate dlts
|
||||||
|
# $ python -m ipykernel install --user --name dlts --display-name "Python (dlts)"
|
||||||
|
|
||||||
|
name: dlts
|
||||||
|
channels:
|
||||||
|
- defaults
|
||||||
|
dependencies:
|
||||||
|
- python==3.6.6
|
||||||
|
- pip>=19.1.1
|
||||||
|
- ipykernel>=4.6.1
|
||||||
|
- jupyter>=1.0.0
|
||||||
|
- matplotlib==3.0.0
|
||||||
|
- numpy==1.16.2
|
||||||
|
- pandas==0.23.4
|
||||||
|
- tensorflow==1.12.0
|
||||||
|
- keras==2.2.4
|
||||||
|
- scikit-learn==0.20.3
|
||||||
|
- statsmodels==0.9.0
|
||||||
|
- xlrd >= 1.0.0
|
||||||
|
- pip:
|
||||||
|
- pyramid-arima==0.8.1
|
@ -0,0 +1,37 @@
|
|||||||
|
import zipfile
|
||||||
|
import os
|
||||||
|
import sys
|
||||||
|
import pandas as pd
|
||||||
|
|
||||||
|
# This function unzips the GEFCom2014 data zip file and extracts the 'extended'
|
||||||
|
# load forecasting competition data. Data is saved in energy.csv
|
||||||
|
def extract_data(data_dir):
|
||||||
|
GEFCom_dir = os.path.join(data_dir, 'GEFCom2014', 'GEFCom2014 Data')
|
||||||
|
|
||||||
|
GEFCom_zipfile = os.path.join(data_dir, 'GEFCom2014.zip')
|
||||||
|
if not os.path.exists(GEFCom_zipfile):
|
||||||
|
sys.exit("Download GEFCom2014.zip from https://www.dropbox.com/s/pqenrr2mcvl0hk9/GEFCom2014.zip?dl=0 and save it to the '{}' directory.".format(data_dir))
|
||||||
|
|
||||||
|
# unzip root directory
|
||||||
|
zip_ref = zipfile.ZipFile(GEFCom_zipfile, 'r')
|
||||||
|
zip_ref.extractall(os.path.join(data_dir, 'GEFCom2014'))
|
||||||
|
zip_ref.close()
|
||||||
|
|
||||||
|
# extract the extended competition data
|
||||||
|
zip_ref = zipfile.ZipFile(os.path.join(GEFCom_dir, 'GEFCom2014-E_V2.zip'), 'r')
|
||||||
|
zip_ref.extractall(os.path.join(data_dir, 'GEFCom2014-E'))
|
||||||
|
zip_ref.close()
|
||||||
|
|
||||||
|
# load the data from Excel file
|
||||||
|
data = pd.read_excel(os.path.join(data_dir, 'GEFCom2014-E', 'GEFCom2014-E.xlsx'), parse_date='Date')
|
||||||
|
|
||||||
|
# create timestamp variable from Date and Hour
|
||||||
|
data['timestamp'] = data['Date'].add(pd.to_timedelta(data.Hour - 1, unit='h'))
|
||||||
|
data = data[['timestamp', 'load', 'T']]
|
||||||
|
data = data.rename(columns={'T':'temp'})
|
||||||
|
|
||||||
|
# remove time period with no load data
|
||||||
|
data = data[data.timestamp >= '2012-01-01']
|
||||||
|
|
||||||
|
# save to csv
|
||||||
|
data.to_csv(os.path.join(data_dir, 'energy.csv'), index=False)
|
@ -0,0 +1,145 @@
|
|||||||
|
import numpy as np
|
||||||
|
import pandas as pd
|
||||||
|
import os
|
||||||
|
from collections import UserDict
|
||||||
|
|
||||||
|
def load_data(data_dir):
|
||||||
|
"""Load the GEFCom 2014 energy load data"""
|
||||||
|
|
||||||
|
energy = pd.read_csv(os.path.join(data_dir, 'energy.csv'), parse_dates=['timestamp'])
|
||||||
|
|
||||||
|
# Reindex the dataframe such that the dataframe has a record for every time point
|
||||||
|
# between the minimum and maximum timestamp in the time series. This helps to
|
||||||
|
# identify missing time periods in the data (there are none in this dataset).
|
||||||
|
|
||||||
|
energy.index = energy['timestamp']
|
||||||
|
energy = energy.reindex(pd.date_range(min(energy['timestamp']),
|
||||||
|
max(energy['timestamp']),
|
||||||
|
freq='H'))
|
||||||
|
energy = energy.drop('timestamp', axis=1)
|
||||||
|
|
||||||
|
return energy
|
||||||
|
|
||||||
|
|
||||||
|
def mape(predictions, actuals):
|
||||||
|
"""Mean absolute percentage error"""
|
||||||
|
return ((predictions - actuals).abs() / actuals).mean()
|
||||||
|
|
||||||
|
|
||||||
|
def create_evaluation_df(predictions, test_inputs, H, scaler):
|
||||||
|
"""Create a data frame for easy evaluation"""
|
||||||
|
eval_df = pd.DataFrame(predictions, columns=['t+'+str(t) for t in range(1, H+1)])
|
||||||
|
eval_df['timestamp'] = test_inputs.dataframe.index
|
||||||
|
eval_df = pd.melt(eval_df, id_vars='timestamp', value_name='prediction', var_name='h')
|
||||||
|
eval_df['actual'] = np.transpose(test_inputs['target']).ravel()
|
||||||
|
eval_df[['prediction', 'actual']] = scaler.inverse_transform(eval_df[['prediction', 'actual']])
|
||||||
|
return eval_df
|
||||||
|
|
||||||
|
|
||||||
|
class TimeSeriesTensor(UserDict):
|
||||||
|
"""A dictionary of tensors for input into the RNN model.
|
||||||
|
|
||||||
|
Use this class to:
|
||||||
|
1. Shift the values of the time series to create a Pandas dataframe containing all the data
|
||||||
|
for a single training example
|
||||||
|
2. Discard any samples with missing values
|
||||||
|
3. Transform this Pandas dataframe into a numpy array of shape
|
||||||
|
(samples, time steps, features) for input into Keras
|
||||||
|
|
||||||
|
The class takes the following parameters:
|
||||||
|
- **dataset**: original time series
|
||||||
|
- **target** name of the target column
|
||||||
|
- **H**: the forecast horizon
|
||||||
|
- **tensor_structures**: a dictionary discribing the tensor structure of the form
|
||||||
|
{ 'tensor_name' : (range(max_backward_shift, max_forward_shift), [feature, feature, ...] ) }
|
||||||
|
if features are non-sequential and should not be shifted, use the form
|
||||||
|
{ 'tensor_name' : (None, [feature, feature, ...])}
|
||||||
|
- **freq**: time series frequency (default 'H' - hourly)
|
||||||
|
- **drop_incomplete**: (Boolean) whether to drop incomplete samples (default True)
|
||||||
|
"""
|
||||||
|
|
||||||
|
def __init__(self, dataset, target, H, tensor_structure, freq='H', drop_incomplete=True):
|
||||||
|
self.dataset = dataset
|
||||||
|
self.target = target
|
||||||
|
self.tensor_structure = tensor_structure
|
||||||
|
self.tensor_names = list(tensor_structure.keys())
|
||||||
|
|
||||||
|
self.dataframe = self._shift_data(H, freq, drop_incomplete)
|
||||||
|
self.data = self._df2tensors(self.dataframe)
|
||||||
|
|
||||||
|
def _shift_data(self, H, freq, drop_incomplete):
|
||||||
|
|
||||||
|
# Use the tensor_structures definitions to shift the features in the original dataset.
|
||||||
|
# The result is a Pandas dataframe with multi-index columns in the hierarchy
|
||||||
|
# tensor - the name of the input tensor
|
||||||
|
# feature - the input feature to be shifted
|
||||||
|
# time step - the time step for the RNN in which the data is input. These labels
|
||||||
|
# are centred on time t. the forecast creation time
|
||||||
|
df = self.dataset.copy()
|
||||||
|
|
||||||
|
idx_tuples = []
|
||||||
|
for t in range(1, H+1):
|
||||||
|
df['t+'+str(t)] = df[self.target].shift(t*-1, freq=freq)
|
||||||
|
idx_tuples.append(('target', 'y', 't+'+str(t)))
|
||||||
|
|
||||||
|
for name, structure in self.tensor_structure.items():
|
||||||
|
rng = structure[0]
|
||||||
|
dataset_cols = structure[1]
|
||||||
|
|
||||||
|
for col in dataset_cols:
|
||||||
|
|
||||||
|
# do not shift non-sequential 'static' features
|
||||||
|
if rng is None:
|
||||||
|
df['context_'+col] = df[col]
|
||||||
|
idx_tuples.append((name, col, 'static'))
|
||||||
|
|
||||||
|
else:
|
||||||
|
for t in rng:
|
||||||
|
sign = '+' if t > 0 else ''
|
||||||
|
shift = str(t) if t != 0 else ''
|
||||||
|
period = 't'+sign+shift
|
||||||
|
shifted_col = name+'_'+col+'_'+period
|
||||||
|
df[shifted_col] = df[col].shift(t*-1, freq=freq)
|
||||||
|
idx_tuples.append((name, col, period))
|
||||||
|
|
||||||
|
df = df.drop(self.dataset.columns, axis=1)
|
||||||
|
idx = pd.MultiIndex.from_tuples(idx_tuples, names=['tensor', 'feature', 'time step'])
|
||||||
|
df.columns = idx
|
||||||
|
|
||||||
|
if drop_incomplete:
|
||||||
|
df = df.dropna(how='any')
|
||||||
|
|
||||||
|
return df
|
||||||
|
|
||||||
|
def _df2tensors(self, dataframe):
|
||||||
|
|
||||||
|
# Transform the shifted Pandas dataframe into the multidimensional numpy arrays. These
|
||||||
|
# arrays can be used to input into the keras model and can be accessed by tensor name.
|
||||||
|
# For example, for a TimeSeriesTensor object named "model_inputs" and a tensor named
|
||||||
|
# "target", the input tensor can be acccessed with model_inputs['target']
|
||||||
|
|
||||||
|
inputs = {}
|
||||||
|
y = dataframe['target']
|
||||||
|
y = y.as_matrix()
|
||||||
|
inputs['target'] = y
|
||||||
|
|
||||||
|
for name, structure in self.tensor_structure.items():
|
||||||
|
rng = structure[0]
|
||||||
|
cols = structure[1]
|
||||||
|
tensor = dataframe[name][cols].as_matrix()
|
||||||
|
if rng is None:
|
||||||
|
tensor = tensor.reshape(tensor.shape[0], len(cols))
|
||||||
|
else:
|
||||||
|
tensor = tensor.reshape(tensor.shape[0], len(cols), len(rng))
|
||||||
|
tensor = np.transpose(tensor, axes=[0, 2, 1])
|
||||||
|
inputs[name] = tensor
|
||||||
|
|
||||||
|
return inputs
|
||||||
|
|
||||||
|
def subset_data(self, new_dataframe):
|
||||||
|
|
||||||
|
# Use this function to recreate the input tensors if the shifted dataframe
|
||||||
|
# has been filtered.
|
||||||
|
|
||||||
|
self.dataframe = new_dataframe
|
||||||
|
self.data = self._df2tensors(self.dataframe)
|
@ -0,0 +1,111 @@
|
|||||||
|
{
|
||||||
|
"cells": [
|
||||||
|
{
|
||||||
|
"cell_type": "code",
|
||||||
|
"execution_count": 2,
|
||||||
|
"metadata": {},
|
||||||
|
"outputs": [],
|
||||||
|
"source": [
|
||||||
|
"import os\n",
|
||||||
|
"import matplotlib.pyplot as plt\n",
|
||||||
|
"from common.utils import load_data\n",
|
||||||
|
"from common.extract_data import extract_data\n",
|
||||||
|
"%matplotlib inline"
|
||||||
|
]
|
||||||
|
},
|
||||||
|
{
|
||||||
|
"cell_type": "code",
|
||||||
|
"execution_count": 3,
|
||||||
|
"metadata": {},
|
||||||
|
"outputs": [
|
||||||
|
{
|
||||||
|
"output_type": "error",
|
||||||
|
"ename": "TypeError",
|
||||||
|
"evalue": "read_excel() got an unexpected keyword argument 'parse_date'",
|
||||||
|
"traceback": [
|
||||||
|
"\u001b[0;31m---------------------------------------------------------------------------\u001b[0m",
|
||||||
|
"\u001b[0;31mTypeError\u001b[0m Traceback (most recent call last)",
|
||||||
|
"\u001b[0;32m<ipython-input-3-370b5c38045d>\u001b[0m in \u001b[0;36m<module>\u001b[0;34m\u001b[0m\n\u001b[1;32m 2\u001b[0m \u001b[0;34m\u001b[0m\u001b[0m\n\u001b[1;32m 3\u001b[0m \u001b[0;32mif\u001b[0m \u001b[0;32mnot\u001b[0m \u001b[0mos\u001b[0m\u001b[0;34m.\u001b[0m\u001b[0mpath\u001b[0m\u001b[0;34m.\u001b[0m\u001b[0mexists\u001b[0m\u001b[0;34m(\u001b[0m\u001b[0mos\u001b[0m\u001b[0;34m.\u001b[0m\u001b[0mpath\u001b[0m\u001b[0;34m.\u001b[0m\u001b[0mjoin\u001b[0m\u001b[0;34m(\u001b[0m\u001b[0mdata_dir\u001b[0m\u001b[0;34m,\u001b[0m \u001b[0;34m'energy.csv'\u001b[0m\u001b[0;34m)\u001b[0m\u001b[0;34m)\u001b[0m\u001b[0;34m:\u001b[0m\u001b[0;34m\u001b[0m\u001b[0m\n\u001b[0;32m----> 4\u001b[0;31m \u001b[0mextract_data\u001b[0m\u001b[0;34m(\u001b[0m\u001b[0mdata_dir\u001b[0m\u001b[0;34m)\u001b[0m\u001b[0;34m\u001b[0m\u001b[0m\n\u001b[0m",
|
||||||
|
"\u001b[0;32m~/Documents/MSFT/curricula/Currriculum-Dev/ml-for-beginners/TimeSeries/1-Introduction/working/common/extract_data.py\u001b[0m in \u001b[0;36mextract_data\u001b[0;34m(data_dir)\u001b[0m\n\u001b[1;32m 24\u001b[0m \u001b[0;34m\u001b[0m\u001b[0m\n\u001b[1;32m 25\u001b[0m \u001b[0;31m# load the data from Excel file\u001b[0m\u001b[0;34m\u001b[0m\u001b[0;34m\u001b[0m\u001b[0m\n\u001b[0;32m---> 26\u001b[0;31m \u001b[0mdata\u001b[0m \u001b[0;34m=\u001b[0m \u001b[0mpd\u001b[0m\u001b[0;34m.\u001b[0m\u001b[0mread_excel\u001b[0m\u001b[0;34m(\u001b[0m\u001b[0mos\u001b[0m\u001b[0;34m.\u001b[0m\u001b[0mpath\u001b[0m\u001b[0;34m.\u001b[0m\u001b[0mjoin\u001b[0m\u001b[0;34m(\u001b[0m\u001b[0mdata_dir\u001b[0m\u001b[0;34m,\u001b[0m \u001b[0;34m'GEFCom2014-E'\u001b[0m\u001b[0;34m,\u001b[0m \u001b[0;34m'GEFCom2014-E.xlsx'\u001b[0m\u001b[0;34m)\u001b[0m\u001b[0;34m,\u001b[0m \u001b[0mparse_date\u001b[0m\u001b[0;34m=\u001b[0m\u001b[0;34m'Date'\u001b[0m\u001b[0;34m)\u001b[0m\u001b[0;34m\u001b[0m\u001b[0m\n\u001b[0m\u001b[1;32m 27\u001b[0m \u001b[0;34m\u001b[0m\u001b[0m\n\u001b[1;32m 28\u001b[0m \u001b[0;31m# create timestamp variable from Date and Hour\u001b[0m\u001b[0;34m\u001b[0m\u001b[0;34m\u001b[0m\u001b[0m\n",
|
||||||
|
"\u001b[0;32m/Library/Frameworks/Python.framework/Versions/3.7/lib/python3.7/site-packages/pandas/util/_decorators.py\u001b[0m in \u001b[0;36mwrapper\u001b[0;34m(*args, **kwargs)\u001b[0m\n\u001b[1;32m 294\u001b[0m )\n\u001b[1;32m 295\u001b[0m \u001b[0mwarnings\u001b[0m\u001b[0;34m.\u001b[0m\u001b[0mwarn\u001b[0m\u001b[0;34m(\u001b[0m\u001b[0mmsg\u001b[0m\u001b[0;34m,\u001b[0m \u001b[0mFutureWarning\u001b[0m\u001b[0;34m,\u001b[0m \u001b[0mstacklevel\u001b[0m\u001b[0;34m=\u001b[0m\u001b[0mstacklevel\u001b[0m\u001b[0;34m)\u001b[0m\u001b[0;34m\u001b[0m\u001b[0m\n\u001b[0;32m--> 296\u001b[0;31m \u001b[0;32mreturn\u001b[0m \u001b[0mfunc\u001b[0m\u001b[0;34m(\u001b[0m\u001b[0;34m*\u001b[0m\u001b[0margs\u001b[0m\u001b[0;34m,\u001b[0m \u001b[0;34m**\u001b[0m\u001b[0mkwargs\u001b[0m\u001b[0;34m)\u001b[0m\u001b[0;34m\u001b[0m\u001b[0m\n\u001b[0m\u001b[1;32m 297\u001b[0m \u001b[0;34m\u001b[0m\u001b[0m\n\u001b[1;32m 298\u001b[0m \u001b[0;32mreturn\u001b[0m \u001b[0mwrapper\u001b[0m\u001b[0;34m\u001b[0m\u001b[0m\n",
|
||||||
|
"\u001b[0;31mTypeError\u001b[0m: read_excel() got an unexpected keyword argument 'parse_date'"
|
||||||
|
]
|
||||||
|
}
|
||||||
|
],
|
||||||
|
"source": [
|
||||||
|
"data_dir = './data'\n",
|
||||||
|
"\n",
|
||||||
|
"if not os.path.exists(os.path.join(data_dir, 'energy.csv')):\n",
|
||||||
|
" extract_data(data_dir)"
|
||||||
|
]
|
||||||
|
},
|
||||||
|
{
|
||||||
|
"cell_type": "code",
|
||||||
|
"execution_count": 4,
|
||||||
|
"metadata": {},
|
||||||
|
"outputs": [
|
||||||
|
{
|
||||||
|
"output_type": "error",
|
||||||
|
"ename": "FileNotFoundError",
|
||||||
|
"evalue": "[Errno 2] No such file or directory: './data/energy.csv'",
|
||||||
|
"traceback": [
|
||||||
|
"\u001b[0;31m---------------------------------------------------------------------------\u001b[0m",
|
||||||
|
"\u001b[0;31mFileNotFoundError\u001b[0m Traceback (most recent call last)",
|
||||||
|
"\u001b[0;32m<ipython-input-4-a4cbf8be04ff>\u001b[0m in \u001b[0;36m<module>\u001b[0;34m\u001b[0m\n\u001b[0;32m----> 1\u001b[0;31m \u001b[0menergy\u001b[0m \u001b[0;34m=\u001b[0m \u001b[0mload_data\u001b[0m\u001b[0;34m(\u001b[0m\u001b[0mdata_dir\u001b[0m\u001b[0;34m)\u001b[0m\u001b[0;34m[\u001b[0m\u001b[0;34m[\u001b[0m\u001b[0;34m'load'\u001b[0m\u001b[0;34m]\u001b[0m\u001b[0;34m]\u001b[0m\u001b[0;34m\u001b[0m\u001b[0m\n\u001b[0m\u001b[1;32m 2\u001b[0m \u001b[0menergy\u001b[0m\u001b[0;34m.\u001b[0m\u001b[0mhead\u001b[0m\u001b[0;34m(\u001b[0m\u001b[0;34m)\u001b[0m\u001b[0;34m\u001b[0m\u001b[0m\n",
|
||||||
|
"\u001b[0;32m~/Documents/MSFT/curricula/Currriculum-Dev/ml-for-beginners/TimeSeries/1-Introduction/working/common/utils.py\u001b[0m in \u001b[0;36mload_data\u001b[0;34m(data_dir)\u001b[0m\n\u001b[1;32m 7\u001b[0m \u001b[0;34m\"\"\"Load the GEFCom 2014 energy load data\"\"\"\u001b[0m\u001b[0;34m\u001b[0m\u001b[0m\n\u001b[1;32m 8\u001b[0m \u001b[0;34m\u001b[0m\u001b[0m\n\u001b[0;32m----> 9\u001b[0;31m \u001b[0menergy\u001b[0m \u001b[0;34m=\u001b[0m \u001b[0mpd\u001b[0m\u001b[0;34m.\u001b[0m\u001b[0mread_csv\u001b[0m\u001b[0;34m(\u001b[0m\u001b[0mos\u001b[0m\u001b[0;34m.\u001b[0m\u001b[0mpath\u001b[0m\u001b[0;34m.\u001b[0m\u001b[0mjoin\u001b[0m\u001b[0;34m(\u001b[0m\u001b[0mdata_dir\u001b[0m\u001b[0;34m,\u001b[0m \u001b[0;34m'energy.csv'\u001b[0m\u001b[0;34m)\u001b[0m\u001b[0;34m,\u001b[0m \u001b[0mparse_dates\u001b[0m\u001b[0;34m=\u001b[0m\u001b[0;34m[\u001b[0m\u001b[0;34m'timestamp'\u001b[0m\u001b[0;34m]\u001b[0m\u001b[0;34m)\u001b[0m\u001b[0;34m\u001b[0m\u001b[0m\n\u001b[0m\u001b[1;32m 10\u001b[0m \u001b[0;34m\u001b[0m\u001b[0m\n\u001b[1;32m 11\u001b[0m \u001b[0;31m# Reindex the dataframe such that the dataframe has a record for every time point\u001b[0m\u001b[0;34m\u001b[0m\u001b[0;34m\u001b[0m\u001b[0m\n",
|
||||||
|
"\u001b[0;32m/Library/Frameworks/Python.framework/Versions/3.7/lib/python3.7/site-packages/pandas/io/parsers.py\u001b[0m in \u001b[0;36mread_csv\u001b[0;34m(filepath_or_buffer, sep, delimiter, header, names, index_col, usecols, squeeze, prefix, mangle_dupe_cols, dtype, engine, converters, true_values, false_values, skipinitialspace, skiprows, skipfooter, nrows, na_values, keep_default_na, na_filter, verbose, skip_blank_lines, parse_dates, infer_datetime_format, keep_date_col, date_parser, dayfirst, cache_dates, iterator, chunksize, compression, thousands, decimal, lineterminator, quotechar, quoting, doublequote, escapechar, comment, encoding, dialect, error_bad_lines, warn_bad_lines, delim_whitespace, low_memory, memory_map, float_precision)\u001b[0m\n\u001b[1;32m 684\u001b[0m )\n\u001b[1;32m 685\u001b[0m \u001b[0;34m\u001b[0m\u001b[0m\n\u001b[0;32m--> 686\u001b[0;31m \u001b[0;32mreturn\u001b[0m \u001b[0m_read\u001b[0m\u001b[0;34m(\u001b[0m\u001b[0mfilepath_or_buffer\u001b[0m\u001b[0;34m,\u001b[0m \u001b[0mkwds\u001b[0m\u001b[0;34m)\u001b[0m\u001b[0;34m\u001b[0m\u001b[0m\n\u001b[0m\u001b[1;32m 687\u001b[0m \u001b[0;34m\u001b[0m\u001b[0m\n\u001b[1;32m 688\u001b[0m \u001b[0;34m\u001b[0m\u001b[0m\n",
|
||||||
|
"\u001b[0;32m/Library/Frameworks/Python.framework/Versions/3.7/lib/python3.7/site-packages/pandas/io/parsers.py\u001b[0m in \u001b[0;36m_read\u001b[0;34m(filepath_or_buffer, kwds)\u001b[0m\n\u001b[1;32m 450\u001b[0m \u001b[0;34m\u001b[0m\u001b[0m\n\u001b[1;32m 451\u001b[0m \u001b[0;31m# Create the parser.\u001b[0m\u001b[0;34m\u001b[0m\u001b[0;34m\u001b[0m\u001b[0m\n\u001b[0;32m--> 452\u001b[0;31m \u001b[0mparser\u001b[0m \u001b[0;34m=\u001b[0m \u001b[0mTextFileReader\u001b[0m\u001b[0;34m(\u001b[0m\u001b[0mfp_or_buf\u001b[0m\u001b[0;34m,\u001b[0m \u001b[0;34m**\u001b[0m\u001b[0mkwds\u001b[0m\u001b[0;34m)\u001b[0m\u001b[0;34m\u001b[0m\u001b[0m\n\u001b[0m\u001b[1;32m 453\u001b[0m \u001b[0;34m\u001b[0m\u001b[0m\n\u001b[1;32m 454\u001b[0m \u001b[0;32mif\u001b[0m \u001b[0mchunksize\u001b[0m \u001b[0;32mor\u001b[0m \u001b[0miterator\u001b[0m\u001b[0;34m:\u001b[0m\u001b[0;34m\u001b[0m\u001b[0m\n",
|
||||||
|
"\u001b[0;32m/Library/Frameworks/Python.framework/Versions/3.7/lib/python3.7/site-packages/pandas/io/parsers.py\u001b[0m in \u001b[0;36m__init__\u001b[0;34m(self, f, engine, **kwds)\u001b[0m\n\u001b[1;32m 934\u001b[0m \u001b[0mself\u001b[0m\u001b[0;34m.\u001b[0m\u001b[0moptions\u001b[0m\u001b[0;34m[\u001b[0m\u001b[0;34m\"has_index_names\"\u001b[0m\u001b[0;34m]\u001b[0m \u001b[0;34m=\u001b[0m \u001b[0mkwds\u001b[0m\u001b[0;34m[\u001b[0m\u001b[0;34m\"has_index_names\"\u001b[0m\u001b[0;34m]\u001b[0m\u001b[0;34m\u001b[0m\u001b[0m\n\u001b[1;32m 935\u001b[0m \u001b[0;34m\u001b[0m\u001b[0m\n\u001b[0;32m--> 936\u001b[0;31m \u001b[0mself\u001b[0m\u001b[0;34m.\u001b[0m\u001b[0m_make_engine\u001b[0m\u001b[0;34m(\u001b[0m\u001b[0mself\u001b[0m\u001b[0;34m.\u001b[0m\u001b[0mengine\u001b[0m\u001b[0;34m)\u001b[0m\u001b[0;34m\u001b[0m\u001b[0m\n\u001b[0m\u001b[1;32m 937\u001b[0m \u001b[0;34m\u001b[0m\u001b[0m\n\u001b[1;32m 938\u001b[0m \u001b[0;32mdef\u001b[0m \u001b[0mclose\u001b[0m\u001b[0;34m(\u001b[0m\u001b[0mself\u001b[0m\u001b[0;34m)\u001b[0m\u001b[0;34m:\u001b[0m\u001b[0;34m\u001b[0m\u001b[0m\n",
|
||||||
|
"\u001b[0;32m/Library/Frameworks/Python.framework/Versions/3.7/lib/python3.7/site-packages/pandas/io/parsers.py\u001b[0m in \u001b[0;36m_make_engine\u001b[0;34m(self, engine)\u001b[0m\n\u001b[1;32m 1166\u001b[0m \u001b[0;32mdef\u001b[0m \u001b[0m_make_engine\u001b[0m\u001b[0;34m(\u001b[0m\u001b[0mself\u001b[0m\u001b[0;34m,\u001b[0m \u001b[0mengine\u001b[0m\u001b[0;34m=\u001b[0m\u001b[0;34m\"c\"\u001b[0m\u001b[0;34m)\u001b[0m\u001b[0;34m:\u001b[0m\u001b[0;34m\u001b[0m\u001b[0m\n\u001b[1;32m 1167\u001b[0m \u001b[0;32mif\u001b[0m \u001b[0mengine\u001b[0m \u001b[0;34m==\u001b[0m \u001b[0;34m\"c\"\u001b[0m\u001b[0;34m:\u001b[0m\u001b[0;34m\u001b[0m\u001b[0m\n\u001b[0;32m-> 1168\u001b[0;31m \u001b[0mself\u001b[0m\u001b[0;34m.\u001b[0m\u001b[0m_engine\u001b[0m \u001b[0;34m=\u001b[0m \u001b[0mCParserWrapper\u001b[0m\u001b[0;34m(\u001b[0m\u001b[0mself\u001b[0m\u001b[0;34m.\u001b[0m\u001b[0mf\u001b[0m\u001b[0;34m,\u001b[0m \u001b[0;34m**\u001b[0m\u001b[0mself\u001b[0m\u001b[0;34m.\u001b[0m\u001b[0moptions\u001b[0m\u001b[0;34m)\u001b[0m\u001b[0;34m\u001b[0m\u001b[0m\n\u001b[0m\u001b[1;32m 1169\u001b[0m \u001b[0;32melse\u001b[0m\u001b[0;34m:\u001b[0m\u001b[0;34m\u001b[0m\u001b[0m\n\u001b[1;32m 1170\u001b[0m \u001b[0;32mif\u001b[0m \u001b[0mengine\u001b[0m \u001b[0;34m==\u001b[0m \u001b[0;34m\"python\"\u001b[0m\u001b[0;34m:\u001b[0m\u001b[0;34m\u001b[0m\u001b[0m\n",
|
||||||
|
"\u001b[0;32m/Library/Frameworks/Python.framework/Versions/3.7/lib/python3.7/site-packages/pandas/io/parsers.py\u001b[0m in \u001b[0;36m__init__\u001b[0;34m(self, src, **kwds)\u001b[0m\n\u001b[1;32m 1996\u001b[0m \u001b[0mkwds\u001b[0m\u001b[0;34m[\u001b[0m\u001b[0;34m\"usecols\"\u001b[0m\u001b[0;34m]\u001b[0m \u001b[0;34m=\u001b[0m \u001b[0mself\u001b[0m\u001b[0;34m.\u001b[0m\u001b[0musecols\u001b[0m\u001b[0;34m\u001b[0m\u001b[0m\n\u001b[1;32m 1997\u001b[0m \u001b[0;34m\u001b[0m\u001b[0m\n\u001b[0;32m-> 1998\u001b[0;31m \u001b[0mself\u001b[0m\u001b[0;34m.\u001b[0m\u001b[0m_reader\u001b[0m \u001b[0;34m=\u001b[0m \u001b[0mparsers\u001b[0m\u001b[0;34m.\u001b[0m\u001b[0mTextReader\u001b[0m\u001b[0;34m(\u001b[0m\u001b[0msrc\u001b[0m\u001b[0;34m,\u001b[0m \u001b[0;34m**\u001b[0m\u001b[0mkwds\u001b[0m\u001b[0;34m)\u001b[0m\u001b[0;34m\u001b[0m\u001b[0m\n\u001b[0m\u001b[1;32m 1999\u001b[0m \u001b[0mself\u001b[0m\u001b[0;34m.\u001b[0m\u001b[0munnamed_cols\u001b[0m \u001b[0;34m=\u001b[0m \u001b[0mself\u001b[0m\u001b[0;34m.\u001b[0m\u001b[0m_reader\u001b[0m\u001b[0;34m.\u001b[0m\u001b[0munnamed_cols\u001b[0m\u001b[0;34m\u001b[0m\u001b[0m\n\u001b[1;32m 2000\u001b[0m \u001b[0;34m\u001b[0m\u001b[0m\n",
|
||||||
|
"\u001b[0;32mpandas/_libs/parsers.pyx\u001b[0m in \u001b[0;36mpandas._libs.parsers.TextReader.__cinit__\u001b[0;34m()\u001b[0m\n",
|
||||||
|
"\u001b[0;32mpandas/_libs/parsers.pyx\u001b[0m in \u001b[0;36mpandas._libs.parsers.TextReader._setup_parser_source\u001b[0;34m()\u001b[0m\n",
|
||||||
|
"\u001b[0;31mFileNotFoundError\u001b[0m: [Errno 2] No such file or directory: './data/energy.csv'"
|
||||||
|
]
|
||||||
|
}
|
||||||
|
],
|
||||||
|
"source": [
|
||||||
|
"energy = load_data(data_dir)[['load']]\n",
|
||||||
|
"energy.head()"
|
||||||
|
]
|
||||||
|
},
|
||||||
|
{
|
||||||
|
"cell_type": "code",
|
||||||
|
"execution_count": null,
|
||||||
|
"metadata": {},
|
||||||
|
"outputs": [],
|
||||||
|
"source": []
|
||||||
|
}
|
||||||
|
],
|
||||||
|
"metadata": {
|
||||||
|
"kernel_info": {
|
||||||
|
"name": "python3"
|
||||||
|
},
|
||||||
|
"kernelspec": {
|
||||||
|
"name": "python37364bit8d3b438fb5fc4430a93ac2cb74d693a7",
|
||||||
|
"display_name": "Python 3.7.0 64-bit ('3.7')"
|
||||||
|
},
|
||||||
|
"language_info": {
|
||||||
|
"codemirror_mode": {
|
||||||
|
"name": "ipython",
|
||||||
|
"version": 3
|
||||||
|
},
|
||||||
|
"file_extension": ".py",
|
||||||
|
"mimetype": "text/x-python",
|
||||||
|
"name": "python",
|
||||||
|
"nbconvert_exporter": "python",
|
||||||
|
"pygments_lexer": "ipython3",
|
||||||
|
"version": "3.7.0"
|
||||||
|
},
|
||||||
|
"nteract": {
|
||||||
|
"version": "nteract-front-end@1.0.0"
|
||||||
|
},
|
||||||
|
"metadata": {
|
||||||
|
"interpreter": {
|
||||||
|
"hash": "70b38d7a306a849643e446cd70466270a13445e5987dfa1344ef2b127438fa4d"
|
||||||
|
}
|
||||||
|
}
|
||||||
|
},
|
||||||
|
"nbformat": 4,
|
||||||
|
"nbformat_minor": 2
|
||||||
|
}
|
Loading…
Reference in new issue