@ -96,7 +96,7 @@ In the near future, understanding the basics of machine learning is going to be
Sketch, on paper or using an online app like [Excalidraw](https://excalidraw.com/), your understanding of the differences between AI, ML, deep learning, and data science. Add some ideas of problems that each of these techniques are good at solving.
@ -98,7 +98,7 @@ Dans un avenir proche, comprendre les bases du machine learning sera indispensab
Esquisser, sur papier ou à l'aide d'une application en ligne comme [Excalidraw](https://excalidraw.com/), votre compréhension des différences entre l'IA, le ML, le deep learning et la data science. Ajouter quelques idées de problèmes que chacune de ces techniques est bonne à résoudre.
## [Quiz de validation des connaissances](https://jolly-sea-0a877260f.azurestaticapps.net/quiz/2?loc=fr)
## [Quiz de validation des connaissances](https://white-water-09ec41f0f.azurestaticapps.net/quiz/2?loc=fr)
@ -96,7 +96,7 @@ Dalam waktu dekat, memahami dasar-dasar Machine Learning akan menjadi suatu keha
Buat sketsa di atas kertas atau menggunakan aplikasi seperti [Excalidraw](https://excalidraw.com/), mengenai pemahaman kamu tentang perbedaan antara AI, ML, Deep Learning, dan Data Science. Tambahkan beberapa ide masalah yang cocok diselesaikan masing-masing teknik.
@ -97,7 +97,7 @@ Nel prossimo futuro, comprendere le basi di machine learning sarà un must per l
Disegnare, su carta o utilizzando un'app online come [Excalidraw](https://excalidraw.com/), la propria comprensione delle differenze tra AI, ML, deep learning e data science. Aggiungere alcune idee sui problemi che ciascuna di queste tecniche è in grado di risolvere.
> 🎥 Makine öğrenimi, yapay zeka ve derin öğrenme arasındaki farkı tartışan bir video için yukarıdaki resme tıklayın.
## [Ders öncesi sınav](https://jolly-sea-0a877260f.azurestaticapps.net/quiz/1?loc=tr)
## [Ders öncesi sınav](https://white-water-09ec41f0f.azurestaticapps.net/quiz/1?loc=tr)
### Introduction
@ -103,7 +103,7 @@ Yakın gelecekte, yaygın olarak benimsenmesi nedeniyle makine öğreniminin tem
Kağıt üzerinde veya [Excalidraw](https://excalidraw.com/) gibi çevrimiçi bir uygulama kullanarak AI, makine öğrenimi, derin öğrenme ve veri bilimi arasındaki farkları anladığınızdan emin olun. Bu tekniklerin her birinin çözmede iyi olduğu bazı problem fikirleri ekleyin.
## [Ders sonrası test](https://jolly-sea-0a877260f.azurestaticapps.net/quiz/2?loc=tr)
## [Ders sonrası test](https://white-water-09ec41f0f.azurestaticapps.net/quiz/2?loc=tr)
In this lesson, we will walk through the major milestones in the history of machine learning and artificial intelligence.
@ -101,7 +101,7 @@ It remains to be seen what the future holds, but it is important to understand t
Dig into one of these historical moments and learn more about the people behind them. There are fascinating characters, and no scientific discovery was ever created in a cultural vacuum. What do you discover?

> Boceto por [Tomomi Imura](https://www.twitter.com/girlie_mac)
## [Cuestionario previo a la conferencia](https://jolly-sea-0a877260f.azurestaticapps.net/quiz/3/)
## [Cuestionario previo a la conferencia](https://white-water-09ec41f0f.azurestaticapps.net/quiz/3/)
En esta lección, analizaremos los principales hitos en la historia del machine learning y la inteligencia artificial.
@ -102,7 +102,7 @@ Queda por ver qué depara el futuro, pero es importante entender estos sistemas
Sumérjase dentro de unos de estos momentos históricos y aprenda más sobre las personas detrás de ellos. Hay personajes fascinantes y nunca se creó ningún descubrimiento científico en un vacío cultural. ¿Qué descubres?
## [Cuestionario posterior a la conferencia](https://jolly-sea-0a877260f.azurestaticapps.net/quiz/4/)
## [Cuestionario posterior a la conferencia](https://white-water-09ec41f0f.azurestaticapps.net/quiz/4/)
Dans cette leçon, nous allons parcourir les principales étapes de l'histoire du machine learning et de l'intelligence artificielle.
@ -102,7 +102,7 @@ Reste à savoir ce que l'avenir nous réserve, mais il est important de comprend
Plongez dans l'un de ces moments historiques et apprenez-en plus sur les personnes derrière ceux-ci. Il y a des personnalités fascinantes, et aucune découverte scientifique n'a jamais été créée avec un vide culturel. Que découvrez-vous ?
## [Quiz de validation des connaissances](https://jolly-sea-0a877260f.azurestaticapps.net/quiz/4?loc=fr)
## [Quiz de validation des connaissances](https://white-water-09ec41f0f.azurestaticapps.net/quiz/4?loc=fr)
Dalam pelajaran ini, kita akan membahas tonggak utama dalam sejarah Machine Learning dan Artificial Intelligence.
@ -101,7 +101,7 @@ Kita masih belum tahu apa yang akan terjadi di masa depan, tetapi penting untuk
Gali salah satu momen bersejarah ini dan pelajari lebih lanjut tentang orang-orang di baliknya. Ada karakter yang menarik, dan tidak ada penemuan ilmiah yang pernah dibuat dalam kekosongan budaya. Apa yang kamu temukan?
In questa lezione, si camminerà attraverso le principali pietre miliari nella storia di machine learning e dell'intelligenza artificiale.
@ -103,7 +103,7 @@ Resta da vedere cosa riserva il futuro, ma è importante capire questi sistemi i
Approfondire uno di questi momenti storici e scoprire
di più sulle persone che stanno dietro ad essi. Ci sono personaggi affascinanti e nessuna scoperta scientifica è mai stata creata in un vuoto culturale. Cosa si è scoperto?
> [Tomomi Imura](https://www.twitter.com/girlie_mac) tarafından hazırlanan taslak-not
## [Ders öncesi test](https://jolly-sea-0a877260f.azurestaticapps.net/quiz/3?loc=tr)
## [Ders öncesi test](https://white-water-09ec41f0f.azurestaticapps.net/quiz/3?loc=tr)
Bu derste, makine öğrenimi ve yapay zeka tarihindeki önemli kilometre taşlarını inceleyeceğiz.
@ -102,7 +102,7 @@ Geleceğin neler getireceğini birlikte göreceğiz, ancak bu bilgisayar sisteml
Bu tarihi anlardan birine girin ve arkasındaki insanlar hakkında daha fazla bilgi edinin. Büyüleyici karakterler var ve kültürel bir boşlukta hiçbir bilimsel keşif yaratılmadı. Ne keşfedersiniz?
## [Ders sonrası test](https://jolly-sea-0a877260f.azurestaticapps.net/quiz/4?loc=tr)
## [Ders sonrası test](https://white-water-09ec41f0f.azurestaticapps.net/quiz/4?loc=tr)
Questa tabella ci dice diverse cose. Innanzitutto, si nota che ci sono relativamente poche persone non binarie nei dati. I dati sono distorti, quindi si deve fare attenzione a come si interpretano questi numeri.
@ -183,7 +183,7 @@ Per evitare che vengano introdotti pregiudizi, in primo luogo, si dovrebbe:
Si pensi a scenari di vita reale in cui l'ingiustizia è evidente nella creazione e nell'utilizzo del modello. Cos'altro si dovrebbe considerare?
@ -103,7 +103,7 @@ In these lessons, you will discover how to use these steps to prepare, build, te
Draw a flow chart reflecting the steps of a ML practitioner. Where do you see yourself right now in the process? Where do you predict you will find difficulty? What seems easy to you?
@ -6,7 +6,7 @@ El proceso de creación, uso y mantenimiento de modelos de machine learning, y l
- Explorar conceptos básicos como 'modelos', 'predicciones', y 'datos de entrenamiento'
## [Cuestionario previo a la conferencia](https://jolly-sea-0a877260f.azurestaticapps.net/quiz/7/)
## [Cuestionario previo a la conferencia](https://white-water-09ec41f0f.azurestaticapps.net/quiz/7/)
## Introducción
A un alto nivel, el arte de crear procesos de machine learning (ML) se compone de una serie de pasos:
@ -101,7 +101,7 @@ En estas lecciones, descubrirá cómo utilizar estos pasos para preparar, constr
Dibuje un diagrama de flujos que refleje los pasos de practicante de ML. ¿Dónde te ves ahora mismo en el proceso? ¿Dónde predice que encontrará dificultades? ¿Qué te parece fácil?
## [Cuestionario posterior a la conferencia](https://jolly-sea-0a877260f.azurestaticapps.net/quiz/8/)
## [Cuestionario posterior a la conferencia](https://white-water-09ec41f0f.azurestaticapps.net/quiz/8/)
Gambaran membuat proses machine learning (ML) terdiri dari sejumlah langkah:
@ -100,7 +100,7 @@ Dalam pelajaran ini, Kamu akan menemukan cara untuk menggunakan langkah-langkah
Gambarlah sebuah flow chart yang mencerminkan langkah-langkah seorang praktisi ML. Di mana kamu melihat diri kamu saat ini dalam prosesnya? Di mana kamu memprediksi kamu akan menemukan kesulitan? Apa yang tampak mudah bagi kamu?
@ -103,7 +103,7 @@ In queste lezioni si scoprirà come utilizzare questi passaggi per preparare, co
Disegnare un diagramma di flusso che rifletta i passaggi di un professionista di ML. Dove ci si vede in questo momento nel processo? Dove si prevede che sorgeranno difficoltà? Cosa sembra facile?
> ### [This lesson is available in R!](./solution/lesson_1-R.ipynb)
@ -199,7 +199,7 @@ Congratulations, you built your first linear regression model, created a predict
## 🚀Challenge
Plot a different variable from this dataset. Hint: edit this line: `X = X[:, np.newaxis, 2]`. Given this dataset's target, what are you able to discover about the progression of diabetes as a disease?
Dalam keempat pelajaran ini, kamu akan belajar bagaimana membangun model regresi. Kita akan berdiskusi apa fungsi model tersebut dalam sejenak. Tetapi sebelum kamu melakukan apapun, pastikan bahwa kamu sudah mempunyai alat-alat yang diperlukan untuk memulai!
@ -195,7 +195,7 @@ Selamat, kamu telah membangun model regresi linear pertamamu, membuat sebuah pre
## Tantangan
Gambarkan sebuah variabel yang beda dari *dataset* ini. Petunjuk: edit baris ini: `X = X[:, np.newaxis, 2]`. Mengetahui target *dataset* ini, apa yang kamu bisa menemukan tentang kemajuan diabetes sebagai sebuah penyakit?
@ -197,7 +197,7 @@ Congratulazioni, si è costruito il primo modello di regressione lineare, creato
Tracciare una variabile diversa da questo insieme di dati. Suggerimento: modificare questa riga: `X = X[:, np.newaxis, 2]`. Dato l'obiettivo di questo insieme di dati, cosa si potrebbe riuscire a scoprire circa la progressione del diabete come matattia?
So far you have explored what regression is with sample data gathered from the pumpkin pricing dataset that we will use throughout this lesson. You have also visualized it using Matplotlib.
@ -320,7 +320,7 @@ It does make sense, given the plot! And, if this is a better model than the prev
Test several different variables in this notebook to see how correlation corresponds to model accuracy.
Selama ini kamu telah menjelajahi apa regresi itu dengan data contoh yang dikumpulkan dari *dataset* harga labu yang kita akan gunakan terus sepanjang pelajaran ini. Kamu juga telah memvisualisasikannya dengan Matplotlib.
@ -324,7 +324,7 @@ Itu sangat masuk akal dengan bagan sebelumnya! Selain itu, jika ini model lebih
Coba-cobalah variabel-variabel yang lain di *notebook* ini untuk melihat bagaimana korelasi berhubungan dengan akurasi model.
@ -222,10 +222,10 @@ While you can get a scoreboard report [terms](https://scikit-learn.org/stable/mo
In Scikit-learn, confusion matrices Rows (axis 0) are actual labels and columns (axis 1) are predicted labels.
| |0|1|
|:-:|:-:|:-:|
|0|TN|FP|
|1|FN|TP|
| |0|1|
|:---:|:---:|:---:|
|0|TN|FP|
|1|FN|TP|
What's going on here? Let's say our model is asked to classify pumpkins between two binary categories, category 'orange' and category 'not-orange'.
@ -296,7 +296,7 @@ In future lessons on classifications, you will learn how to iterate to improve y
There's a lot more to unpack regarding logistic regression! But the best way to learn is to experiment. Find a dataset that lends itself to this type of analysis and build a model with it. What do you learn? tip: try [Kaggle](https://www.kaggle.com/search?q=logistic+regression+datasets) for interesting datasets.
@ -291,7 +291,7 @@ Nanti dalam pelajaran lebih lanjut tentang klasifikasi, kamu akan belajar bagaim
Masih ada banyak tentang regresi logistik! Tetapi cara paling baik adalah untuk bereksperimen. Carilah sebuah *dataset* yang bisa diteliti seperti ini dan bangunlah sebuah model darinya. Apa yang kamu pelajari? Petunjuk: Coba [Kaggle](https://kaggle.com) untuk *dataset-dataset* menarik.
@ -284,7 +284,7 @@ Nelle lezioni future sulle classificazioni si imparerà come eseguire l'iterazio
C'è molto altro da svelare riguardo alla regressione logistica! Ma il modo migliore per imparare è sperimentare. Trovare un insieme di dati che si presti a questo tipo di analisi e costruire un modello con esso. Cosa si è appreso? suggerimento: provare [Kaggle](https://kaggle.com) per ottenere insiemi di dati interessanti.
@ -334,7 +334,7 @@ In a professional setting, you can see how good communication is necessary betwe
Instead of working in a notebook and importing the model to the Flask app, you could train the model right within the Flask app! Try converting your Python code in the notebook, perhaps after your data is cleaned, to train the model from within the app on a route called `train`. What are the pros and cons of pursuing this method?
@ -334,7 +334,7 @@ In un ambiente professionale, si può vedere quanto sia necessaria una buona com
Invece di lavorare su un notebook e importare il modello nell'app Flask, si può addestrare il modello direttamente nell'app Flask! Provare a convertire il codice Python nel notebook, magari dopo che i dati sono stati puliti, per addestrare il modello dall'interno dell'app su un percorso chiamato `/train`. Quali sono i pro e i contro nel seguire questo metodo?
Classification uses various algorithms to determine other ways of determining a data point's label or class. Let's work with this cuisine data to see whether, by observing a group of ingredients, we can determine its cuisine of origin.
@ -286,7 +286,7 @@ Now that you have cleaned the data, use [SMOTE](https://imbalanced-learn.org/dev
This curriculum contains several interesting datasets. Dig through the `data` folders and see if any contain datasets that would be appropriate for binary or multi-class classification? What questions would you ask of this dataset?
La classificazione utilizza vari algoritmi per determinare altri modi per definire l'etichetta o la classe di un punto dati. Si lavorerà con questi dati di cucina per vedere se, osservando un gruppo di ingredienti, è possibile determinarne la cucina di origine.
@ -286,7 +286,7 @@ Ora che i dati sono puliti, si usa [SMOTE](https://imbalanced-learn.org/dev/refe
Questo programma di studi contiene diversi insiemi di dati interessanti. Esaminare le cartelle `data` e vedere se contiene insiemi di dati che sarebbero appropriati per la classificazione binaria o multiclasse. Quali domande si farebbero a questo insieme di dati?
Sınıflandırma, bir veri noktasının etiketini veya sınıfını belirlemek için farklı yollar belirlemek üzere çeşitli algoritmalar kullanır. Bir grup malzemeyi gözlemleyerek kökeninin hangi mutfak olduğunu belirleyip belirleyemeyeceğimizi görmek için bu mutfak verisiyle çalışalım.
## [Ders öncesi kısa sınavı](https://jolly-sea-0a877260f.azurestaticapps.net/quiz/19/?loc=tr)
## [Ders öncesi kısa sınavı](https://white-water-09ec41f0f.azurestaticapps.net/quiz/19/?loc=tr)
### Giriş
@ -287,7 +287,7 @@ Veriyi temizlediniz, şimdi [SMOTE](https://imbalanced-learn.org/dev/references/
Bu öğretim programı farklı ilgi çekici veri setleri içermekte. `data` klasörlerini inceleyin ve ikili veya çok sınıflı sınıflandırma için uygun olabilecek veri setleri bulunduran var mı, bakın. Bu veri seti için hangi soruları sorabilirdiniz?
## [Ders sonrası kısa sınavı](https://jolly-sea-0a877260f.azurestaticapps.net/quiz/20/?loc=tr)
## [Ders sonrası kısa sınavı](https://white-water-09ec41f0f.azurestaticapps.net/quiz/20/?loc=tr)
@ -4,7 +4,7 @@ In this lesson, you will use the dataset you saved from the last lesson full of
You will use this dataset with a variety of classifiers to _predict a given national cuisine based on a group of ingredients_. While doing so, you'll learn more about some of the ways that algorithms can be leveraged for classification tasks.
Assuming you completed [Lesson 1](../1-Introduction/README.md), make sure that a _cleaned_cuisines.csv_ file exists in the root `/data` folder for these four lessons.
@ -67,13 +67,13 @@ Assuming you completed [Lesson 1](../1-Introduction/README.md), make sure that a
In this lesson, you used your cleaned data to build a machine learning model that can predict a national cuisine based on a series of ingredients. Take some time to read through the many options Scikit-learn provides to classify data. Dig deeper into the concept of 'solver' to understand what goes on behind the scenes.
@ -4,7 +4,7 @@ In questa lezione, si utilizzerà l'insieme di dati salvati dall'ultima lezione,
Si utilizzerà questo insieme di dati con una varietà di classificatori per _prevedere una determinata cucina nazionale in base a un gruppo di ingredienti_. Mentre si fa questo, si imparerà di più su alcuni dei modi in cui gli algoritmi possono essere sfruttati per le attività di classificazione.
Supponendo che la [Lezione 1](../1-Introduction/README.md) sia stata completata, assicurarsi che _esista_ un file clean_cuisines.csv nella cartella in radice `/data` per queste quattro lezioni.
@ -200,13 +200,13 @@ Poiché si sta utilizzando il caso multiclasse, si deve scegliere quale _schema_
Il risultato è stampato: la cucina indiana è la sua ipotesi migliore, con buone probabilità:
In questa lezione, sono stati utilizzati dati puliti per creare un modello di apprendimento automatico in grado di prevedere una cucina nazionale basata su una serie di ingredienti. Si prenda del tempo per leggere le numerose opzioni fornite da Scikit-learn per classificare i dati. Approfondire il concetto di "risolutore" per capire cosa succede dietro le quinte.
Approfondire un po' la matematica alla base della regressione logistica in [questa lezione](https://people.eecs.berkeley.edu/~russell/classes/cs194/f11/lectures/CS194%20Fall%202011%20Lecture%2006.pdf)
@ -4,7 +4,7 @@ Bu derste, mutfaklarla ilgili dengeli ve temiz veriyle dolu, geçen dersten kayd
Bu veri setini çeşitli sınıflandırıcılarla _bir grup malzemeyi baz alarak verilen bir ulusal mutfağı öngörmek_ için kullanacaksınız. Bunu yaparken, sınıflandırma görevleri için algoritmaların leveraj edilebileceği yollardan bazıları hakkında daha fazla bilgi edineceksiniz.
## [Ders öncesi kısa sınavı](https://jolly-sea-0a877260f.azurestaticapps.net/quiz/21/?loc=tr)
## [Ders öncesi kısa sınavı](https://white-water-09ec41f0f.azurestaticapps.net/quiz/21/?loc=tr)
# Hazırlık
[Birinci dersi](../../1-Introduction/README.md) tamamladığınızı varsayıyoruz, dolayısıyla bu dört ders için _cleaned_cuisines.csv_ dosyasının kök `/data` klasöründe var olduğundan emin olun.
@ -69,11 +69,11 @@ Bu veri setini çeşitli sınıflandırıcılarla _bir grup malzemeyi baz alarak
Bu derste, bir grup malzemeyi baz alarak bir ulusal mutfağı öngörebilen bir makine öğrenimi modeli oluşturmak için temiz verinizi kullandınız. Scikit-learn'ün veri sınıflandırmak için sağladığı birçok yöntemi okumak için biraz vakit ayırın. Arka tarafta neler olduğunu anlamak için 'çözücü' kavramını derinlemesine inceleyin.
## [Ders sonrası kısa sınavı](https://jolly-sea-0a877260f.azurestaticapps.net/quiz/22/?loc=tr)
## [Ders sonrası kısa sınavı](https://white-water-09ec41f0f.azurestaticapps.net/quiz/22/?loc=tr)
In this second classification lesson, you will explore more ways to classify numeric data. You will also learn about the ramifications for choosing one classifier over the other.
@ -224,7 +224,7 @@ This method of Machine Learning "combines the predictions of several base estima
Each of these techniques has a large number of parameters that you can tweak. Research each one's default parameters and think about what tweaking these parameters would mean for the model's quality.
In questa seconda lezione sulla classificazione, si esploreranno più modi per classificare i dati numerici. Si Impareranno anche le ramificazioni per la scelta di un classificatore rispetto all'altro.
@ -224,7 +224,7 @@ Questo metodo di Machine Learning "combina le previsioni di diversi stimatori di
Ognuna di queste tecniche ha un gran numero di parametri che si possono modificare. Ricercare i parametri predefiniti di ciascuno e pensare a cosa significherebbe modificare questi parametri per la qualità del modello.
Bu ikinci sınıflandırma dersinde, sayısal veriyi sınıflandırmak için daha fazla yöntem öğreneceksiniz. Ayrıca, bir sınıflandırıcıyı diğerlerine tercih etmenin sonuçlarını da öğreneceksiniz.
## [Ders öncesi kısa sınavı](https://jolly-sea-0a877260f.azurestaticapps.net/quiz/23/?loc=tr)
## [Ders öncesi kısa sınavı](https://white-water-09ec41f0f.azurestaticapps.net/quiz/23/?loc=tr)
### Ön koşul
@ -224,7 +224,7 @@ Makine Öğreniminin bu yöntemi, modelin kalitesini artırmak için, "birçok t
Bu yöntemlerden her biri değiştirebileceğiniz birsürü parametre içeriyor. Her birinin varsayılan parametrelerini araştırın ve bu parametreleri değiştirmenin modelin kalitesi için ne anlama gelebileceği hakkında düşünün.
## [Ders sonrası kısa sınavı](https://jolly-sea-0a877260f.azurestaticapps.net/quiz/24/?loc=tr)
## [Ders sonrası kısa sınavı](https://white-water-09ec41f0f.azurestaticapps.net/quiz/24/?loc=tr)
@ -321,7 +321,7 @@ Congratulations, you have created a 'recommendation' web app with a few fields.
Your web app is very minimal, so continue to build it out using ingredients and their indexes from the [ingredient_indexes](../data/ingredient_indexes.csv) data. What flavor combinations work to create a given national dish?
@ -321,7 +321,7 @@ Congratulazioni, si è creato un'app web di "raccomandazione" con pochi campi. S
L'app web è molto minimale, quindi continuare a costruirla usando gli ingredienti e i loro indici dai dati [ingredient_indexes](../../data/ingredient_indexes.csv) . Quali combinazioni di sapori funzionano per creare un determinato piatto nazionale?
@ -8,7 +8,7 @@ Makine öğreniminin en faydalı pratik kullanımlarından biri, önerici/tavsiy
> :movie_camera: Video için yukarıdaki fotoğrafa tıklayın: Andrew Ng introduces recommendation system design (Andrew Ng önerici sistem tasarımını tanıtıyor)
## [Ders öncesi kısa sınavı](https://jolly-sea-0a877260f.azurestaticapps.net/quiz/25/?loc=tr)
## [Ders öncesi kısa sınavı](https://white-water-09ec41f0f.azurestaticapps.net/quiz/25/?loc=tr)
Bu derste şunları öğreneceksiniz:
@ -321,7 +321,7 @@ Tebrikler, birkaç değişkenle bir 'önerici' web uygulaması oluşturdunuz! Bu
Web uygulamanız çok minimal, bu yüzden [ingredient_indexes](../../data/ingredient_indexes.csv) verisinden malzemeleri ve indexlerini kullanarak web uygulamanızı oluşturmaya devam edin. Verilen bir ulusal yemeği yapmak için hangi tat birleşimleri işe yarıyor?
## [Ders sonrası kısa sınavı](https://jolly-sea-0a877260f.azurestaticapps.net/quiz/26/?loc=tr)
## [Ders sonrası kısa sınavı](https://white-water-09ec41f0f.azurestaticapps.net/quiz/26/?loc=tr)
@ -5,7 +5,7 @@ Clustering is a type of [Unsupervised Learning](https://wikipedia.org/wiki/Unsup
[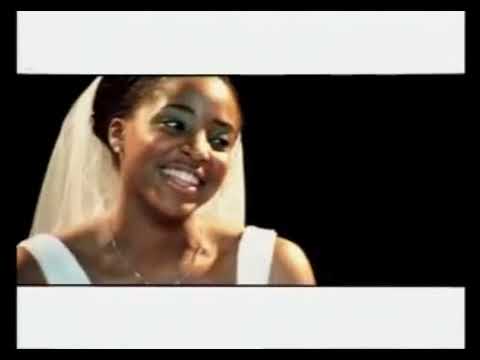](https://youtu.be/ty2advRiWJM "No One Like You by PSquare")
> 🎥 Click the image above for a video. While you're studying machine learning with clustering, enjoy some Nigerian Dance Hall tracks - this is a highly rated song from 2014 by PSquare.
[Clustering](https://link.springer.com/referenceworkentry/10.1007%2F978-0-387-30164-8_124) is very useful for data exploration. Let's see if it can help discover trends and patterns in the way Nigerian audiences consume music.
@ -317,7 +317,7 @@ In general, for clustering, you can use scatterplots to show clusters of data, s
In preparation for the next lesson, make a chart about the various clustering algorithms you might discover and use in a production environment. What kinds of problems is the clustering trying to address?
@ -5,7 +5,7 @@ Il clustering è un tipo di [apprendimento non supervisionato](https://wikipedia
[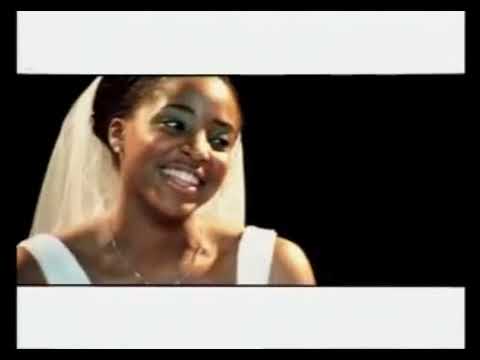](https://youtu.be/ty2advRiWJM "No One Like You di PSquare")
> 🎥 Fare clic sull'immagine sopra per un video. Mentre si studia machine learning con il clustering, si potranno gradire brani della Nigerian Dance Hall: questa è una canzone molto apprezzata del 2014 di PSquare.
@ -33,18 +33,18 @@ In questo [modulo di apprendimento](https://docs.microsoft.com/learn/modules/tra
[Scikit-learn offre una vasta gamma](https://scikit-learn.org/stable/modules/clustering.html) di metodi per eseguire il clustering. Il tipo scelto dipenderà dal caso d'uso. Secondo la documentazione, ogni metodo ha diversi vantaggi. Ecco una tabella semplificata dei metodi supportati da Scikit-learn e dei loro casi d'uso appropriati:
> 🤔 Se si sta lavorando con il clustering, un metodo non supervisionato che non richiede dati etichettati, perché si stanno mostrando questi dati con etichette? Nella fase di esplorazione dei dati, sono utili, ma non sono necessari per il funzionamento degli algoritmi di clustering. Si potrebbero anche rimuovere le intestazioni delle colonne e fare riferimento ai dati per numero di colonna.
@ -319,7 +319,7 @@ In generale, per il clustering è possibile utilizzare i grafici a dispersione p
In preparazione per la lezione successiva, creare un grafico sui vari algoritmi di clustering che si potrebbero scoprire e utilizzare in un ambiente di produzione. Che tipo di problemi sta cercando di affrontare il clustering?
In this lesson, you will learn how to create clusters using Scikit-learn and the Nigerian music dataset you imported earlier. We will cover the basics of K-Means for Clustering. Keep in mind that, as you learned in the earlier lesson, there are many ways to work with clusters and the method you use depends on your data. We will try K-Means as it's the most common clustering technique. Let's get started!
@ -238,7 +238,7 @@ Spend some time with this notebook, tweaking parameters. Can you improve the acc
Hint: Try to scale your data. There's commented code in the notebook that adds standard scaling to make the data columns resemble each other more closely in terms of range. You'll find that while the silhouette score goes down, the 'kink' in the elbow graph smooths out. This is because leaving the data unscaled allows data with less variance to carry more weight. Read a bit more on this problem [here](https://stats.stackexchange.com/questions/21222/are-mean-normalization-and-feature-scaling-needed-for-k-means-clustering/21226#21226).
In questa lezione si imparerà come creare cluster utilizzando Scikit-learn e l'insieme di dati di musica nigeriana importato in precedenza. Si tratteranno le basi di K-Means per Clustering. Si tenga presente che, come appreso nella lezione precedente, ci sono molti modi per lavorare con i cluster e il metodo usato dipende dai propri dati. Si proverà K-Means poiché è la tecnica di clustering più comune. Si inizia!
@ -238,7 +238,7 @@ Trascorrere un po' di tempo con questo notebook, modificando i parametri. E poss
Suggerimento: provare a ridimensionare i dati. C'è un codice commentato nel notebook che aggiunge il ridimensionamento standard per rendere le colonne di dati più simili tra loro in termini di intervallo. Si scoprirà che mentre il punteggio della silhouette diminuisce, il "kink" nel grafico del gomito si attenua. Questo perché lasciare i dati non scalati consente ai dati con meno varianza di avere più peso. Leggere un po' di più su questo problema [qui](https://stats.stackexchange.com/questions/21222/are-mean-normalization-and-feature-scaling-needed-for-k-means-clustering/21226#21226).
Questa lezione copre una breve storia e concetti importanti dell' *elaborazione del linguaggio naturale*, un sottocampo della *linguistica computazionale*.
For most *natural language processing* tasks, the text to be processed, must be broken down, examined, and the results stored or cross referenced with rules and data sets. These tasks, allows the programmer to derive the _meaning_ or _intent_ or only the _frequency_ of terms and words in a text.
Let's discover common techniques used in processing text. Combined with machine learning, these techniques help you to analyse large amounts of text efficiently. Before applying ML to these tasks, however, let's understand the problems encountered by an NLP specialist.
@ -203,7 +203,7 @@ Implement the bot in the prior knowledge check and test it on a friend. Can it t
Take a task in the prior knowledge check and try to implement it. Test the bot on a friend. Can it trick them? Can you make your bot more 'believable?'
Per la maggior parte delle attività di *elaborazione del linguaggio naturale* , il testo da elaborare deve essere suddiviso, esaminato e i risultati archiviati o incrociati con regole e insiemi di dati. Queste attività consentono al programmatore di derivare il _significato_ o l'_intento_ o solo la _frequenza_ di termini e parole in un testo.
Si esaminano le comuni tecniche utilizzate nell'elaborazione del testo. Combinate con machine learning, queste tecniche aiutano ad analizzare grandi quantità di testo in modo efficiente. Prima di applicare machine learning a queste attività, tuttavia, occorre cercare di comprendere i problemi incontrati da uno specialista in NLP.
@ -203,7 +203,7 @@ Implementare il bot nel controllo delle conoscenze precedenti e testarlo su un a
Prendere un'attività dalla verifica delle conoscenze qui sopra e provare a implementarla. Provare il bot su un amico. Può ingannarlo? Si può rendere il bot più 'credibile?'
In the previous lessons you learned how to build a basic bot using `TextBlob`, a library that embeds ML behind-the-scenes to perform basic NLP tasks such as noun phrase extraction. Another important challenge in computational linguistics is accurate _translation_ of a sentence from one spoken or written language to another.
Translation is a very hard problem compounded by the fact that there are thousands of languages and each can have very different grammar rules. One approach is to convert the formal grammar rules for one language, such as English, into a non-language dependent structure, and then translate it by converting back to another language. This approach means that you would take the following steps:
@ -176,7 +176,7 @@ Here is a sample [solution](solution/notebook.ipynb).
Can you make Marvin even better by extracting other features from the user input?
Nelle lezioni precedenti si è imparato come creare un bot di base utilizzando `TextBlob`, una libreria che incorpora machine learning dietro le quinte per eseguire attività di base di NPL come l'estrazione di frasi nominali. Un'altra sfida importante nella linguistica computazionale è _la traduzione_ accurata di una frase da una lingua parlata o scritta a un'altra.
La traduzione è un problema molto difficile, aggravato dal fatto che ci sono migliaia di lingue e ognuna può avere regole grammaticali molto diverse. Un approccio consiste nel convertire le regole grammaticali formali per una lingua, come l'inglese, in una struttura non dipendente dalla lingua e quindi tradurla convertendola in un'altra lingua. Questo approccio significa che si dovrebbero eseguire i seguenti passaggi:
@ -176,7 +176,7 @@ Ecco una [soluzione](../solution/notebook.ipynb) di esempio.
Si può rendere Marvin ancora migliore estraendo altre funzionalità dall'input dell'utente?
@ -393,7 +393,7 @@ Now that you have explored the dataset, in the next lesson you will filter the d
This lesson demonstrates, as we saw in previous lessons, how critically important it is to understand your data and its foibles before performing operations on it. Text-based data, in particular, bears careful scrutiny. Dig through various text-heavy datasets and see if you can discover areas that could introduce bias or skewed sentiment into a model.
| Britannia International Hotel Canary Wharf | 9086 | 4789 |
| Park Plaza Westminster Bridge Londra | 12158 | 4169 |
| Copthorne Tara Hotel London Kensington | 7105 | 3578 |
| ... | ... | ... |
| Mercure Paris Porte d'Orléans | 110 | 10 |
| Hotel Wagner | 135 | 10 |
| Hotel Gallitzinberg | 173 | 8 |
Si potrebbe notare che il *conteggio nell'insieme di dati* non corrisponde al valore in `Total_Number_of_Reviews`. Non è chiaro se questo valore nell'insieme di dati rappresentasse il numero totale di recensioni che l'hotel aveva, ma non tutte sono state recuperate o qualche altro calcolo. `Total_Number_of_Reviews` non viene utilizzato nel modello a causa di questa non chiarezza.
@ -324,19 +324,19 @@ Trattare le seguenti domande come attività di codifica e provare a rispondere s
Ci si potrebbe anche chiedere del valore `Average_Score` e perché a volte è diverso dal punteggio medio calcolato. Poiché non è possibile sapere perché alcuni valori corrispondano, ma altri hanno una differenza, in questo caso è più sicuro utilizzare i punteggi delle recensioni a disposizione per calcolare autonomamente la media. Detto questo, le differenze sono solitamente molto piccole, ecco gli hotel con la maggiore deviazione dalla media dell'insieme di dati e dalla media calcolata:
Con un solo hotel con una differenza di punteggio maggiore di 1, significa che probabilmente si può ignorare la differenza e utilizzare il punteggio medio calcolato.
@ -401,7 +401,7 @@ Ora che si è esplorato l'insieme di dati, nella prossima lezione si filtreranno
Questa lezione dimostra, come visto nelle lezioni precedenti, quanto sia di fondamentale importanza comprendere i dati e le loro debolezze prima di eseguire operazioni su di essi. I dati basati su testo, in particolare, sono oggetto di un attento esame. Esaminare vari insiemi di dati contenenti principalmente testo e vedere se si riesce a scoprire aree che potrebbero introdurre pregiudizi o sentiment distorti in un modello.
Now that you have a explored the dataset in detail, it's time to filter the columns and then use NLP techniques on the dataset to gain new insights about the hotels.
When you started, you had a dataset with columns and data but not all of it could be verified or used. You've explored the data, filtered out what you don't need, converted tags into something useful, calculated your own averages, added some sentiment columns and hopefully, learned some interesting things about processing natural text.
Ora che si è esplorato in dettaglio l'insieme di dati, è il momento di filtrare le colonne e quindi utilizzare le tecniche NLP sull'insieme di dati per ottenere nuove informazioni sugli hotel.
2. Elaborazione colonne di meta-recensione dell'hotel
@ -361,7 +361,7 @@ Per riepilogare, i passaggi sono:
Quando si è iniziato, si disponeva di un insieme di dati con colonne e dati, ma non tutto poteva essere verificato o utilizzato. Si sono esplorati i dati, filtrato ciò che non serve, convertito i tag in qualcosa di utile, calcolato le proprie medie, aggiunto alcune colonne di sentiment e, si spera, imparato alcune cose interessanti sull'elaborazione del testo naturale.
It's a useful and interesting field with real value to business, given its direct application to problems of pricing, inventory, and supply chain issues. While deep learning techniques have started to be used to gain more insights to better predict future performance, time series forecasting remains a field greatly informed by classic ML techniques.
@ -174,7 +174,7 @@ In the next lesson, you will create an ARIMA model to create some forecasts.
Make a list of all the industries and areas of inquiry you can think of that would benefit from time series forecasting. Can you think of an application of these techniques in the arts? In Econometrics? Ecology? Retail? Industry? Finance? Where else?
@ -383,7 +383,7 @@ Check the accuracy of your model by testing its mean absolute percentage error (
Dig into the ways to test the accuracy of a Time Series Model. We touch on MAPE in this lesson, but are there other methods you could use? Research them and annotate them. A helpful document can be found [here](https://otexts.com/fpp2/accuracy.html)
@ -314,6 +314,6 @@ The learnings can be summarized as:
Overall, it is important to remember that the success and quality of the learning process significantly depends on parameters, such as learning rate, learning rate decay, and discount factor. Those are often called **hyperparameters**, to distinguish them from **parameters**, which we optimize during training (for example, Q-Table coefficients). The process of finding the best hyperparameter values is called **hyperparameter optimization**, and it deserves a separate topic.
The problem we have been solving in the previous lesson might seem like a toy problem, not really applicable for real life scenarios. This is not the case, because many real world problems also share this scenario - including playing Chess or Go. They are similar, because we also have a board with given rules and a **discrete state**.
@ -329,7 +329,7 @@ You should see something like this:
> **Task 4**: Here we were not selecting the best action on each step, but rather sampling with corresponding probability distribution. Would it make more sense to always select the best action, with the highest Q-Table value? This can be done by using `np.argmax` function to find out the action number corresponding to highers Q-Table value. Implement this strategy and see if it improves the balancing.
@ -8,7 +8,7 @@ In this curriculum, you have learned many ways to prepare data for training and
While a lot of interest in industry has been garnered by AI, which usually leverages deep learning, there are still valuable applications for classical machine learning models. You might even use some of these applications today! In this lesson, you'll explore how eight different industries and subject-matter domains use these types of models to make their applications more performant, reliable, intelligent, and valuable to users.
@ -26,7 +26,7 @@ Similar to Readme's, please translate the assignments as well.
3. Edit the quiz-app's [translations index.js file](https://github.com/microsoft/ML-For-Beginners/blob/main/quiz-app/src/assets/translations/index.js) to add your language.
4. Finally, edit ALL the quiz links in your translated README.md files to point directly to your translated quiz: https://https://jolly-sea-0a877260f.azurestaticapps.net/quiz/1 becomes https://jolly-sea-0a877260f.azurestaticapps.net/quiz/1?loc=id
4. Finally, edit ALL the quiz links in your translated README.md files to point directly to your translated quiz: https://white-water-09ec41f0f.azurestaticapps.net/quiz/1 becomes https://white-water-09ec41f0f.azurestaticapps.net/quiz/1?loc=id