In these four lessons, you will discover how to build regression models. We will discuss what these are for shortly. But before you do anything, make sure you have the right tools in place to start the process!
- Configure your computer for local machine learning tasks.
- 为本地机器学习任务配置你的计算机。
- Work with Jupyter notebooks.
- 使用Jupyter notebooks。
- Use Scikit-learn, including installation.
- 使用Scikit-learn,包括安装。
- Explore linear regression with a hands-on exercise.
- 通过动手练习探索线性回归。
## Installations and configurations
## 安装和配置
[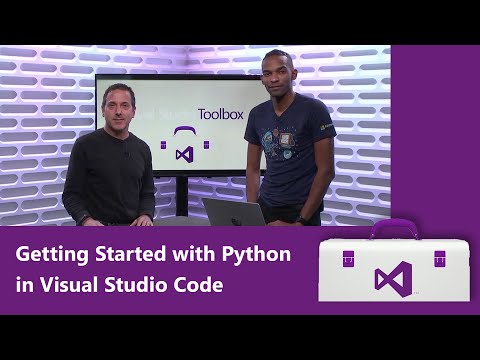](https://youtu.be/7EXd4_ttIuw "Using Python with Visual Studio Code")
[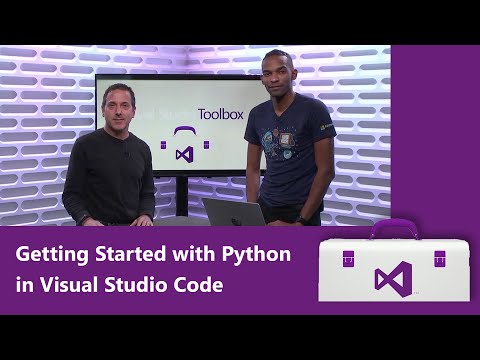](https://youtu.be/7EXd4_ttIuw "在 Visual Studio Code中使用 Python")
> 🎥 Click the image above for a video: using Python within VS Code.
> 🎥 单击上图观看视频:在VS Code中使用Python。
1. **Install Python**. Ensure that [Python](https://www.python.org/downloads/) is installed on your computer. You will use Python for many data science and machine learning tasks. Most computer systems already include a Python installation. There are useful [Python Coding Packs](https://code.visualstudio.com/learn/educators/installers?WT.mc_id=academic-15963-cxa) available as well, to ease the setup for some users.
Some usages of Python, however, require one version of the software, whereas others require a different version. For this reason, it's useful to work within a [virtual environment](https://docs.python.org/3/library/venv.html).
2. **Install Visual Studio Code**. Make sure you have Visual Studio Code installed on your computer. Follow these instructions to [install Visual Studio Code](https://code.visualstudio.com/) for the basic installation. You are going to use Python in Visual Studio Code in this course, so you might want to brush up on how to [configure Visual Studio Code](https://docs.microsoft.com/learn/modules/python-install-vscode?WT.mc_id=academic-15963-cxa) for Python development.
2. **安装 Visual Studio Code**。确保你的计算机上安装了Visual Studio Code。按照这些说明[安装 Visual Studio Code](https://code.visualstudio.com/)进行基本安装。在本课程中,你将在Visual Studio Code中使用Python,因此你可能想复习如何[配置 Visual Studio Code](https://docs.microsoft.com/learn/modules/python-install-vscode?WT.mc_id=academic-15963-cxa)用于Python开发。
> Get comfortable with Python by working through this collection of [Learn modules](https://docs.microsoft.com/users/jenlooper-2911/collections/mp1pagggd5qrq7?WT.mc_id=academic-15963-cxa)
3. **Install Scikit-learn**, by following [these instructions](https://scikit-learn.org/stable/install.html). Since you need to ensure that you use Python 3, it's recommended that you use a virtual environment. Note, if you are installing this library on a M1 Mac, there are special instructions on the page linked above.
You are going to use **notebooks** to develop your Python code and create machine learning models. This type of file is a common tool for data scientists, and they can be identified by their suffix or extension `.ipynb`.
Notebooks are an interactive environment that allow the developer to both code and add notes and write documentation around the code which is quite helpful for experimental or research-oriented projects.
A Jupyter server will start with Python 3+ started. You will find areas of the notebook that can be `run`, pieces of code. You can run a code block, by selecting the icon that looks like a play button.
2. 选择`md`图标并添加一点markdown,输入文字**#Welcome to your notebook**。
1. Select the `md` icon and add a bit of markdown, and the following text **# Welcome to your notebook**.
接下来,添加一些Python代码。
Next, add some Python code.
1. 在代码块中输入**print("hello notebook")**。
1. Type **print('hello notebook')** in the code block.
2. 选择箭头运行代码。
1. Select the arrow to run the code.
You should see the printed statement:
你应该看到打印的语句:
```output
```output
hello notebook
hello notebook
```
```


You can interleaf your code with comments to self-document the notebook.
你可以为你的代码添加注释,以便notebook可以自描述。
✅ Think for a minute how different a web developer's working environment is versus that of a data scientist.
✅ 想一想web开发人员的工作环境与数据科学家的工作环境有多大的不同。
## Up and running with Scikit-learn
## 启动并运行Scikit-learn
Now that Python is set up in your local environment, and you are comfortable with Jupyter notebooks, let's get equally comfortable with Scikit-learn (pronounce it `sci` as in `science`). Scikit-learn provides an [extensive API](https://scikit-learn.org/stable/modules/classes.html#api-ref) to help you perform ML tasks.
According to their [website](https://scikit-learn.org/stable/getting_started.html), "Scikit-learn is an open source machine learning library that supports supervised and unsupervised learning. It also provides various tools for model fitting, data preprocessing, model selection and evaluation, and many other utilities."
In this course, you will use Scikit-learn and other tools to build machine learning models to perform what we call 'traditional machine learning' tasks. We have deliberately avoided neural networks and deep learning, as they are better covered in our forthcoming 'AI for Beginners' curriculum.
Scikit-learn makes it straightforward to build models and evaluate them for use. It is primarily focused on using numeric data and contains several ready-made datasets for use as learning tools. It also includes pre-built models for students to try. Let's explore the process of loading prepackaged data and using a built in estimator first ML model with Scikit-learn with some basic data.
Scikit-learn使构建模型和评估它们的使用变得简单。它主要侧重于使用数字数据,并包含几个现成的数据集用作学习工具。它还包括供学生尝试的预建模型。让我们探索加载预先打包的数据和使用内置的estimator first ML模型和Scikit-learn以及一些基本数据的过程。
## Exercise - your first Scikit-learn notebook
## 练习 - 你的第一个Scikit-learn notebook
> This tutorial was inspired by the [linear regression example](https://scikit-learn.org/stable/auto_examples/linear_model/plot_ols.html#sphx-glr-auto-examples-linear-model-plot-ols-py) on Scikit-learn's web site.
In the _notebook.ipynb_ file associated to this lesson, clear out all the cells by pressing the 'trash can' icon.
在与本课程相关的_notebook.ipynb_文件中,通过点击“垃圾桶”图标清除所有单元格。
In this section, you will work with a small dataset about diabetes that is built into Scikit-learn for learning purposes. Imagine that you wanted to test a treatment for diabetic patients. Machine Learning models might help you determine which patients would respond better to the treatment, based on combinations of variables. Even a very basic regression model, when visualized, might show information about variables that would help you organize your theoretical clinical trials.
✅ There are many types of regression methods, and which one you pick depends on the answer you're looking for. If you want to predict the probable height for a person of a given age, you'd use linear regression, as you're seeking a **numeric value**. If you're interested in discovering whether a type of cuisine should be considered vegan or not, you're looking for a **category assignment** so you would use logistic regression. You'll learn more about logistic regression later. Think a bit about some questions you can ask of data, and which of these methods would be more appropriate.
@ -107,26 +106,26 @@ Import some libraries to help with your tasks.
from sklearn import datasets, linear_model, model_selection
from sklearn import datasets, linear_model, model_selection
```
```
Above you are importing `matplottlib`, `numpy` and you are importing `datasets`, `linear_model` and `model_selection` from `sklearn`. `model_selection` is used for splitting data into training and test sets.
The built-in [diabetes dataset](https://scikit-learn.org/stable/datasets/toy_dataset.html#diabetes-dataset) includes 442 samples of data around diabetes, with 10 feature variables, some of which include:
✅ This dataset includes the concept of 'sex' as a feature variable important to research around diabetes. Many medical datasets include this type of binary classification. Think a bit about how categorizations such as this might exclude certain parts of a population from treatments.
> 🎓 Remember, this is supervised learning, and we need a named 'y' target.
> 🎓 请记住,这是监督学习,我们需要一个命名为“y”的目标。
In a new code cell, load the diabetes dataset by calling `load_diabetes()`. The input `return_X_y=True` signals that `X` will be a data matrix, and `y` will be the regression target.
1. Add some print commands to show the shape of the data matrix and its first element:
1. 添加一些打印命令来显示数据矩阵的形状及其第一个元素:
```python
```python
X, y = datasets.load_diabetes(return_X_y=True)
X, y = datasets.load_diabetes(return_X_y=True)
@ -134,9 +133,9 @@ In a new code cell, load the diabetes dataset by calling `load_diabetes()`. The
print(X[0])
print(X[0])
```
```
What you are getting back as a response, is a tuple. What you are doing is to assign the two first values of the tuple to `X` and `y` respectively. Learn more [about tuples](https://wikipedia.org/wiki/Tuple).
You can see that this data has 442 items shaped in arrays of 10 elements:
你可以看到这个数据有442个项目,组成了10个元素的数组:
```text
```text
(442, 10)
(442, 10)
@ -144,38 +143,38 @@ In a new code cell, load the diabetes dataset by calling `load_diabetes()`. The
-0.04340085 -0.00259226 0.01990842 -0.01764613]
-0.04340085 -0.00259226 0.01990842 -0.01764613]
```
```
✅ Think a bit about the relationship between the data and the regression target. Linear regression predicts relationships between feature X and target variable y. Can you find the [target](https://scikit-learn.org/stable/datasets/toy_dataset.html#diabetes-dataset) for the diabetes dataset in the documentation? What is this dataset demonstrating, given that target?
2. Next, select a portion of this dataset to plot by arranging it into a new array using numpy's `newaxis` function. We are going to use linear regression to generate a line between values in this data, according to a pattern it determines.
✅ At any time, print out the data to check its shape.
✅ 随时打印数据以检查其形状。
3. Now that you have data ready to be plotted, you can see if a machine can help determine a logical split between the numbers in this dataset. To do this, you need to split both the data (X) and the target (y) into test and training sets. Scikit-learn has a straightforward way to do this; you can split your test data at a given point.
✅ `model.fit()` is a function you'll see in many ML libraries such as TensorFlow
✅ `model.fit()`是一个你会在许多机器学习库(例如 TensorFlow)中看到的函数
5. Then, create a prediction using test data, using the function `predict()`. This will be used to draw the line between data groups
5. 然后,使用函数`predict()`,使用测试数据创建预测。这将用于绘制数据组之间的线
```python
```python
y_pred = model.predict(X_test)
y_pred = model.predict(X_test)
```
```
6. Now it's time to show the data in a plot. Matplotlib is a very useful tool for this task. Create a scatterplot of all the X and y test data, and use the prediction to draw a line in the most appropriate place, between the model's data groupings.
@ -183,24 +182,24 @@ In a new code cell, load the diabetes dataset by calling `load_diabetes()`. The
plt.show()
plt.show()
```
```


✅ Think a bit about what's going on here. A straight line is running through many small dots of data, but what is it doing exactly? Can you see how you should be able to use this line to predict where a new, unseen data point should fit in relationship to the plot's y axis? Try to put into words the practical use of this model.
Congratulations, you built your first linear regression model, created a prediction with it, and displayed it in a plot!
恭喜,你构建了第一个线性回归模型,使用它创建了预测,并将其显示在绘图中!
---
---
## 🚀Challenge
## 🚀挑战
Plot a different variable from this dataset. Hint: edit this line: `X = X[:, np.newaxis, 2]`. Given this dataset's target, what are you able to discover about the progression of diabetes as a disease?
In this tutorial, you worked with simple linear regression, rather than univariate or multiple linear regression. Read a little about the differences between these methods, or take a look at [this video](https://www.coursera.org/lecture/quantifying-relationships-regression-models/linear-vs-nonlinear-categorical-variables-ai2Ef)
Read more about the concept of regression and think about what kinds of questions can be answered by this technique. Take this [tutorial](https://docs.microsoft.com/learn/modules/train-evaluate-regression-models?WT.mc_id=academic-15963-cxa) to deepen your understanding.