Clustering is a type of [Unsupervised Learning](https://wikipedia.org/wiki/Unsupervised_learning) that presumes that a dataset is unlabelled. It uses various algorithms to sort through unlabeled data and provide groupings according to patterns it discerns in the data.
[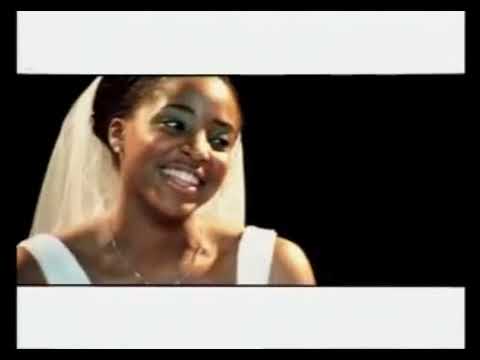](https://youtu.be/ty2advRiWJM "No One Like You by PSquare")
> 🎥 Click the image above for a video. While you're studying Machine Learning with Clustering, enjoy some Nigerian Dance Hall tracks - this is a highly rated song from 2014 by PSquare.
> 🎥 Click the image above for a video. While you're studying machine learning with clustering, enjoy some Nigerian Dance Hall tracks - this is a highly rated song from 2014 by PSquare.
[Clustering](https://link.springer.com/referenceworkentry/10.1007%2F978-0-387-30164-8_124) is very useful for data exploration. Let's see if it can help discover trends and patterns in the way Nigerian audiences consume music.
✅ Take a minute to think about the uses of clustering. In real life, clustering happens whenever you have a pile of laundry and need to sort out your family members' clothes 🧦👕👖🩲. In data science, clustering happens when trying to analyze a user's preferences, or determine the characteristics of any unlabeled dataset. Clustering, in a way, helps make sense of chaos.
✅ Take a minute to think about the uses of clustering. In real life, clustering happens whenever you have a pile of laundry and need to sort out your family members' clothes 🧦👕👖🩲. In data science, clustering happens when trying to analyze a user's preferences, or determine the characteristics of any unlabeled dataset. Clustering, in a way, helps make sense of chaos, like a sock drawer.
[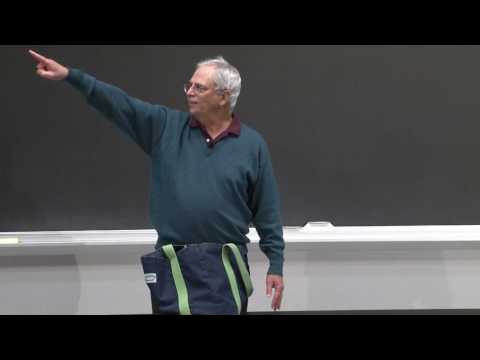](https://youtu.be/esmzYhuFnds "Introduction to Clustering")
> 🎥 Click the image above for a video: MIT's John Guttag introduces Clustering
> 🎥 Click the image above for a video: MIT's John Guttag introduces clustering
In a professional setting, clustering can be used to determine things like market segmentation, determining what age groups buy what items, for example. Another use would be anomaly detection, perhaps to detect fraud from a dataset of credit card transactions. Or you might use clustering to determine tumors in a batch of medical scans.
✅ Think a minute about how you might have encountered clustering 'in the wild', in a banking, e-commerce, or business setting.
> 🎓 Interestingly, Cluster Analysis originated in the fields of Anthropology and Psychology in the 1930s. Can you imagine how it might have been used?
> 🎓 Interestingly, Cluster analysis originated in the fields of Anthropology and Psychology in the 1930s. Can you imagine how it might have been used?
Alternately, you could use it for grouping search results - by shopping links, images, or reviews, for example. Clustering is useful when you have a large dataset that you want to reduce and on which you want to perform more granular analysis, so the technique can be used to learn about data before other models are constructed.
✅ Once your data is organized in clusters, you assign it a cluster Id, and this technique can be useful when preserving a dataset's privacy; you can instead refer to a data point by its cluster id, rather than by more revealing identifiable data. Can you think of other reasons why you'd refer to a cluster Id rather than other elements of the cluster to identify it?
Deepen your understanding of Clustering techniques in this [Learn module](https://docs.microsoft.com/learn/modules/train-evaluate-cluster-models?WT.mc_id=academic-15963-cxa)
Deepen your understanding of clustering techniques in this [Learn module](https://docs.microsoft.com/learn/modules/train-evaluate-cluster-models?WT.mc_id=academic-15963-cxa)
## Getting started with clustering
[Scikit-learn offers a large array](https://scikit-learn.org/stable/modules/clustering.html) of methods to perform clustering. The type you choose will depend on your use case. According to the documentation, each method has various benefits. Here is a simplified table of the methods supported by Scikit-learn and their appropriate use cases:
@ -75,13 +75,13 @@ Deepen your understanding of Clustering techniques in this [Learn module](https:
>
> Data that is 'noisy' is considered to be 'dense'. The distances between points in each of its clusters may prove, on examination, to be more or less dense, or 'crowded' and thus this data needs to be analyzed with the appropriate clustering method. [This article](https://www.kdnuggets.com/2020/02/understanding-density-based-clustering.html) demonstrates the difference between using K-Means clustering vs. HDBSCAN algorithms to explore a noisy dataset with uneven cluster density.
### Clustering Algorithms
### Clustering algorithms
There are over 100 clustering algorithms, and their use depends on the nature of the data at hand. Let's discuss some of the major ones:
**Hierarchical clustering**
If an object is classified by its proximity to a nearby object, rather than to one farther away, clusters are formed based on their members' distance to and from other objects. Scikit-learn's Agglomerative clustering is hierarchical.
If an object is classified by its proximity to a nearby object, rather than to one farther away, clusters are formed based on their members' distance to and from other objects. Scikit-learn's agglomerative clustering is hierarchical.
> Infographic by [Dasani Madipalli](https://twitter.com/dasani_decoded)
@ -95,7 +95,7 @@ This popular algorithm requires the choice of 'k', or the number of clusters to
**Distribution-based clustering**
Based in statistical modeling, distribution-based clustering centers on determining the probability that a data point belongs to a cluster, and assigning it accordingly. Gaussian Mixture methods belong to this type.
Based in statistical modeling, distribution-based clustering centers on determining the probability that a data point belongs to a cluster, and assigning it accordingly. Gaussian mixture methods belong to this type.
**Density-based clustering**
@ -120,7 +120,7 @@ Append the song data .csv file. Load up a dataframe with some data about the son
import matplotlib.pyplot as plt
import pandas as pd
df = pd.read_csv("../../data/nigerian-songs.csv")
df = pd.read_csv("../data/nigerian-songs.csv")
df.head()
```
@ -271,7 +271,7 @@ Is there any convergence in this dataset around a song's perceived popularity an
✅ Try different datapoints (energy, loudness, speechiness) and more or different musical genres. What can you discover? Take a look at the `df.describe()` table to see the general spread of the data points.
### Data Distribution
### Data distribution
Are these three genres significantly different in the perception of their danceability, based on their popularity? Examine our top three genres data distribution for popularity and danceability along a given x and y axis.
@ -289,7 +289,7 @@ You can discover concentric circles around a general point of convergence, showi
> 🎓 Note that this example uses a KDE (Kernel Density Estimate) graph that represents the data using a continuous probability density curve. This allows us to interpret data when working with multiple distributions.
In general, the three genres align loosely in terms of their popularity and danceability. Determining clusters in this loosely-aligned data will be interesting:
In general, the three genres align loosely in terms of their popularity and danceability. Determining clusters in this loosely-aligned data will be a challenge:
In general, for clustering, you can use scatterplots to show clusters of data, so mastering this type of visualization is very useful. In the next lesson, we will take this filtered data and use k-means clustering to discover groups in this data that seems to overlap in interesting ways.
In general, for clustering, you can use scatterplots to show clusters of data, so mastering this type of visualization is very useful. In the next lesson, we will take this filtered data and use k-means clustering to discover groups in this data that see to overlap in interesting ways.
---
## 🚀Challenge
In preparation for the next lesson, make a chart about the various clustering algorithms you might discover and use in a production environment. What kinds of problems is the clustering trying to address?