Merge branch 'main' of https://github.com/microsoft/IoT-For-Beginners into main
commit
7afed015f4
@ -0,0 +1,16 @@
|
||||
# Iniciando con IoT
|
||||
|
||||
En esta sección del curso, serás introducido al Internet de las cosas y aprenderás los conceptos básicos, incluyendo el desarrollo de tu primer proyecto “Hola Mundo” conectado a la nube. Este proyecto consiste en una lampara que se enciende según los niveles de iluminación medidos por un sensor.
|
||||
|
||||
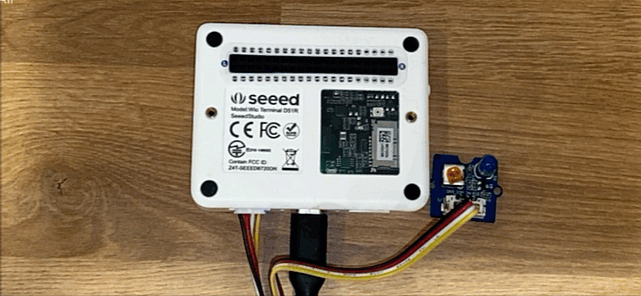
|
||||
|
||||
## Lecciones
|
||||
|
||||
1. [Introducción al IoT](lessons/1-introduction-to-iot/README.md)
|
||||
1. [IoT a profundidad](lessons/2-deeper-dive/README.md)
|
||||
1. [Interactúa con el mundo físico con sensores y actuadores](lessons/3-sensors-and-actuators/README.md)
|
||||
1. [Conecta tu dispositivo al Internet](lessons/4-connect-internet/README.md)
|
||||
|
||||
## Créditos
|
||||
|
||||
Todas las lecciones fueron escritas con ♥️ por [Jim Bennett](https://GitHub.com/JimBobBennett)
|
@ -1,9 +0,0 @@
|
||||
# Dummy File
|
||||
|
||||
This file acts as a placeholder for the `translations` folder. <br>
|
||||
**Please remove this file after adding the first translation**
|
||||
|
||||
For the instructions, follow the directives in the [translations guide](https://github.com/microsoft/IoT-For-Beginners/blob/main/TRANSLATIONS.md) .
|
||||
|
||||
## THANK YOU
|
||||
We truly appreciate your efforts!
|
@ -1,9 +1,10 @@
|
||||
import time
|
||||
import seeed_si114x
|
||||
from grove.grove_light_sensor_v1_2 import GroveLightSensor
|
||||
|
||||
light_sensor = seeed_si114x.grove_si114x()
|
||||
light_sensor = GroveLightSensor(0)
|
||||
|
||||
while True:
|
||||
light = light_sensor.ReadVisible
|
||||
light = light_sensor.light
|
||||
print('Light level:', light)
|
||||
|
||||
time.sleep(1)
|
@ -0,0 +1,5 @@
|
||||
.pio
|
||||
.vscode/.browse.c_cpp.db*
|
||||
.vscode/c_cpp_properties.json
|
||||
.vscode/launch.json
|
||||
.vscode/ipch
|
@ -0,0 +1,7 @@
|
||||
{
|
||||
// See http://go.microsoft.com/fwlink/?LinkId=827846
|
||||
// for the documentation about the extensions.json format
|
||||
"recommendations": [
|
||||
"platformio.platformio-ide"
|
||||
]
|
||||
}
|
@ -0,0 +1,39 @@
|
||||
|
||||
This directory is intended for project header files.
|
||||
|
||||
A header file is a file containing C declarations and macro definitions
|
||||
to be shared between several project source files. You request the use of a
|
||||
header file in your project source file (C, C++, etc) located in `src` folder
|
||||
by including it, with the C preprocessing directive `#include'.
|
||||
|
||||
```src/main.c
|
||||
|
||||
#include "header.h"
|
||||
|
||||
int main (void)
|
||||
{
|
||||
...
|
||||
}
|
||||
```
|
||||
|
||||
Including a header file produces the same results as copying the header file
|
||||
into each source file that needs it. Such copying would be time-consuming
|
||||
and error-prone. With a header file, the related declarations appear
|
||||
in only one place. If they need to be changed, they can be changed in one
|
||||
place, and programs that include the header file will automatically use the
|
||||
new version when next recompiled. The header file eliminates the labor of
|
||||
finding and changing all the copies as well as the risk that a failure to
|
||||
find one copy will result in inconsistencies within a program.
|
||||
|
||||
In C, the usual convention is to give header files names that end with `.h'.
|
||||
It is most portable to use only letters, digits, dashes, and underscores in
|
||||
header file names, and at most one dot.
|
||||
|
||||
Read more about using header files in official GCC documentation:
|
||||
|
||||
* Include Syntax
|
||||
* Include Operation
|
||||
* Once-Only Headers
|
||||
* Computed Includes
|
||||
|
||||
https://gcc.gnu.org/onlinedocs/cpp/Header-Files.html
|
@ -0,0 +1,46 @@
|
||||
|
||||
This directory is intended for project specific (private) libraries.
|
||||
PlatformIO will compile them to static libraries and link into executable file.
|
||||
|
||||
The source code of each library should be placed in a an own separate directory
|
||||
("lib/your_library_name/[here are source files]").
|
||||
|
||||
For example, see a structure of the following two libraries `Foo` and `Bar`:
|
||||
|
||||
|--lib
|
||||
| |
|
||||
| |--Bar
|
||||
| | |--docs
|
||||
| | |--examples
|
||||
| | |--src
|
||||
| | |- Bar.c
|
||||
| | |- Bar.h
|
||||
| | |- library.json (optional, custom build options, etc) https://docs.platformio.org/page/librarymanager/config.html
|
||||
| |
|
||||
| |--Foo
|
||||
| | |- Foo.c
|
||||
| | |- Foo.h
|
||||
| |
|
||||
| |- README --> THIS FILE
|
||||
|
|
||||
|- platformio.ini
|
||||
|--src
|
||||
|- main.c
|
||||
|
||||
and a contents of `src/main.c`:
|
||||
```
|
||||
#include <Foo.h>
|
||||
#include <Bar.h>
|
||||
|
||||
int main (void)
|
||||
{
|
||||
...
|
||||
}
|
||||
|
||||
```
|
||||
|
||||
PlatformIO Library Dependency Finder will find automatically dependent
|
||||
libraries scanning project source files.
|
||||
|
||||
More information about PlatformIO Library Dependency Finder
|
||||
- https://docs.platformio.org/page/librarymanager/ldf.html
|
@ -0,0 +1,16 @@
|
||||
; PlatformIO Project Configuration File
|
||||
;
|
||||
; Build options: build flags, source filter
|
||||
; Upload options: custom upload port, speed and extra flags
|
||||
; Library options: dependencies, extra library storages
|
||||
; Advanced options: extra scripting
|
||||
;
|
||||
; Please visit documentation for the other options and examples
|
||||
; https://docs.platformio.org/page/projectconf.html
|
||||
|
||||
[env:seeed_wio_terminal]
|
||||
platform = atmelsam
|
||||
board = seeed_wio_terminal
|
||||
framework = arduino
|
||||
lib_deps =
|
||||
seeed-studio/Grove Ranging sensor - VL53L0X @ ^1.1.1
|
@ -0,0 +1,31 @@
|
||||
#include <Arduino.h>
|
||||
#include "Seeed_vl53l0x.h"
|
||||
|
||||
Seeed_vl53l0x VL53L0X;
|
||||
|
||||
void setup()
|
||||
{
|
||||
Serial.begin(9600);
|
||||
|
||||
while (!Serial)
|
||||
; // Wait for Serial to be ready
|
||||
|
||||
delay(1000);
|
||||
|
||||
VL53L0X.VL53L0X_common_init();
|
||||
VL53L0X.VL53L0X_high_accuracy_ranging_init();
|
||||
}
|
||||
|
||||
void loop()
|
||||
{
|
||||
VL53L0X_RangingMeasurementData_t RangingMeasurementData;
|
||||
memset(&RangingMeasurementData, 0, sizeof(VL53L0X_RangingMeasurementData_t));
|
||||
|
||||
VL53L0X.PerformSingleRangingMeasurement(&RangingMeasurementData);
|
||||
|
||||
Serial.print("Distance = ");
|
||||
Serial.print(RangingMeasurementData.RangeMilliMeter);
|
||||
Serial.println(" mm");
|
||||
|
||||
delay(1000);
|
||||
}
|
@ -0,0 +1,11 @@
|
||||
|
||||
This directory is intended for PlatformIO Unit Testing and project tests.
|
||||
|
||||
Unit Testing is a software testing method by which individual units of
|
||||
source code, sets of one or more MCU program modules together with associated
|
||||
control data, usage procedures, and operating procedures, are tested to
|
||||
determine whether they are fit for use. Unit testing finds problems early
|
||||
in the development cycle.
|
||||
|
||||
More information about PlatformIO Unit Testing:
|
||||
- https://docs.platformio.org/page/plus/unit-testing.html
|
@ -1,5 +1,20 @@
|
||||
# Consumer IoT - build a smart voice assistant
|
||||
|
||||
The fod has been grown, driven to a processing plant, sorted for quality, sold in the store and now it's time to cook! One of the core pieces of any kitchen is a timer. Initially these started as hour glasses - your food was cooked when all the sand trickled down into the bottom bulb. They then went clockwork, then electric.
|
||||
|
||||
The latest iterations are now part of our smart devices. In kitchens in homes all throughout the world you'll hear cooks shouting "Hey Siri - set a 10 minute timer", or "Alexa - cancel my bread timer". No longer do you have to walk back to the kitchen to check on a timer, you can do it from your phone, or a call out across the room.
|
||||
|
||||
In these 4 lessons you'll learn how to build a smart timer, using AI to recognize your voice, understand what you are asking for, and reply with information about your timer. You'll also add support for multiple languages.
|
||||
|
||||
> 💁 These lessons will use some cloud resources. If you don't complete all the lessons in this project, make sure you [Clean up your project](../clean-up.md).
|
||||
|
||||
## Topics
|
||||
|
||||
1. [Recognize speech with an IoT device](./lessons/1-speech-recognition/README.md)
|
||||
1. [Understand language](./lessons/2-language-understanding/README.md)
|
||||
1. [Set a timer and provide spoken feedback](./lessons/3-spoken-feedback/README.md)
|
||||
1. [Support multiple languages](./lessons/4-multiple-language-support/README.md)
|
||||
|
||||
## Credits
|
||||
|
||||
All the lessons were written with ♥️ by [Jim Bennett](https://GitHub.com/JimBobBennett)
|
||||
|
@ -0,0 +1,9 @@
|
||||
#
|
||||
|
||||
## Instructions
|
||||
|
||||
## Rubric
|
||||
|
||||
| Criteria | Exemplary | Adequate | Needs Improvement |
|
||||
| -------- | --------- | -------- | ----------------- |
|
||||
| | | | |
|
@ -0,0 +1,93 @@
|
||||
import io
|
||||
import json
|
||||
import pyaudio
|
||||
import requests
|
||||
import time
|
||||
import wave
|
||||
|
||||
from azure.iot.device import IoTHubDeviceClient, Message
|
||||
|
||||
from grove.factory import Factory
|
||||
button = Factory.getButton('GPIO-HIGH', 5)
|
||||
|
||||
audio = pyaudio.PyAudio()
|
||||
microphone_card_number = 1
|
||||
speaker_card_number = 1
|
||||
rate = 48000
|
||||
|
||||
def capture_audio():
|
||||
stream = audio.open(format = pyaudio.paInt16,
|
||||
rate = rate,
|
||||
channels = 1,
|
||||
input_device_index = microphone_card_number,
|
||||
input = True,
|
||||
frames_per_buffer = 4096)
|
||||
|
||||
frames = []
|
||||
|
||||
while button.is_pressed():
|
||||
frames.append(stream.read(4096))
|
||||
|
||||
stream.stop_stream()
|
||||
stream.close()
|
||||
|
||||
wav_buffer = io.BytesIO()
|
||||
with wave.open(wav_buffer, 'wb') as wavefile:
|
||||
wavefile.setnchannels(1)
|
||||
wavefile.setsampwidth(audio.get_sample_size(pyaudio.paInt16))
|
||||
wavefile.setframerate(rate)
|
||||
wavefile.writeframes(b''.join(frames))
|
||||
wav_buffer.seek(0)
|
||||
|
||||
return wav_buffer
|
||||
|
||||
api_key = '<key>'
|
||||
location = '<location>'
|
||||
language = '<language>'
|
||||
connection_string = '<connection_string>'
|
||||
|
||||
device_client = IoTHubDeviceClient.create_from_connection_string(connection_string)
|
||||
|
||||
print('Connecting')
|
||||
device_client.connect()
|
||||
print('Connected')
|
||||
|
||||
def get_access_token():
|
||||
headers = {
|
||||
'Ocp-Apim-Subscription-Key': api_key
|
||||
}
|
||||
|
||||
token_endpoint = f'https://{location}.api.cognitive.microsoft.com/sts/v1.0/issuetoken'
|
||||
response = requests.post(token_endpoint, headers=headers)
|
||||
return str(response.text)
|
||||
|
||||
def convert_speech_to_text(buffer):
|
||||
url = f'https://{location}.stt.speech.microsoft.com/speech/recognition/conversation/cognitiveservices/v1'
|
||||
|
||||
headers = {
|
||||
'Authorization': 'Bearer ' + get_access_token(),
|
||||
'Content-Type': f'audio/wav; codecs=audio/pcm; samplerate={rate}',
|
||||
'Accept': 'application/json;text/xml'
|
||||
}
|
||||
|
||||
params = {
|
||||
'language': language
|
||||
}
|
||||
|
||||
response = requests.post(url, headers=headers, params=params, data=buffer)
|
||||
response_json = json.loads(response.text)
|
||||
|
||||
if response_json['RecognitionStatus'] == 'Success':
|
||||
return response_json['DisplayText']
|
||||
else:
|
||||
return ''
|
||||
|
||||
while True:
|
||||
while not button.is_pressed():
|
||||
time.sleep(.1)
|
||||
|
||||
buffer = capture_audio()
|
||||
text = convert_speech_to_text(buffer)
|
||||
if len(text) > 0:
|
||||
message = Message(json.dumps({ 'speech': text }))
|
||||
device_client.send_message(message)
|
@ -0,0 +1,33 @@
|
||||
import json
|
||||
import time
|
||||
from azure.cognitiveservices.speech import SpeechConfig, SpeechRecognizer
|
||||
from azure.iot.device import IoTHubDeviceClient, Message
|
||||
|
||||
api_key = '<key>'
|
||||
location = '<location>'
|
||||
language = '<language>'
|
||||
connection_string = '<connection_string>'
|
||||
|
||||
device_client = IoTHubDeviceClient.create_from_connection_string(connection_string)
|
||||
|
||||
print('Connecting')
|
||||
device_client.connect()
|
||||
print('Connected')
|
||||
|
||||
speech_config = SpeechConfig(subscription=api_key,
|
||||
region=location,
|
||||
speech_recognition_language=language)
|
||||
|
||||
recognizer = SpeechRecognizer(speech_config=speech_config)
|
||||
|
||||
def recognized(args):
|
||||
if len(args.result.text) > 0:
|
||||
message = Message(json.dumps({ 'speech': args.result.text }))
|
||||
device_client.send_message(message)
|
||||
|
||||
recognizer.recognized.connect(recognized)
|
||||
|
||||
recognizer.start_continuous_recognition()
|
||||
|
||||
while True:
|
||||
time.sleep(1)
|
@ -0,0 +1,61 @@
|
||||
import io
|
||||
import pyaudio
|
||||
import time
|
||||
import wave
|
||||
|
||||
from grove.factory import Factory
|
||||
button = Factory.getButton('GPIO-HIGH', 5)
|
||||
|
||||
audio = pyaudio.PyAudio()
|
||||
microphone_card_number = 1
|
||||
speaker_card_number = 1
|
||||
rate = 48000
|
||||
|
||||
def capture_audio():
|
||||
stream = audio.open(format = pyaudio.paInt16,
|
||||
rate = rate,
|
||||
channels = 1,
|
||||
input_device_index = microphone_card_number,
|
||||
input = True,
|
||||
frames_per_buffer = 4096)
|
||||
|
||||
frames = []
|
||||
|
||||
while button.is_pressed():
|
||||
frames.append(stream.read(4096))
|
||||
|
||||
stream.stop_stream()
|
||||
stream.close()
|
||||
|
||||
wav_buffer = io.BytesIO()
|
||||
with wave.open(wav_buffer, 'wb') as wavefile:
|
||||
wavefile.setnchannels(1)
|
||||
wavefile.setsampwidth(audio.get_sample_size(pyaudio.paInt16))
|
||||
wavefile.setframerate(rate)
|
||||
wavefile.writeframes(b''.join(frames))
|
||||
wav_buffer.seek(0)
|
||||
|
||||
return wav_buffer
|
||||
|
||||
def play_audio(buffer):
|
||||
stream = audio.open(format = pyaudio.paInt16,
|
||||
rate = rate,
|
||||
channels = 1,
|
||||
output_device_index = speaker_card_number,
|
||||
output = True)
|
||||
|
||||
with wave.open(buffer, 'rb') as wf:
|
||||
data = wf.readframes(4096)
|
||||
|
||||
while len(data) > 0:
|
||||
stream.write(data)
|
||||
data = wf.readframes(4096)
|
||||
|
||||
stream.close()
|
||||
|
||||
while True:
|
||||
while not button.is_pressed():
|
||||
time.sleep(.1)
|
||||
|
||||
buffer = capture_audio()
|
||||
play_audio(buffer)
|
@ -0,0 +1,82 @@
|
||||
import io
|
||||
import json
|
||||
import pyaudio
|
||||
import requests
|
||||
import time
|
||||
import wave
|
||||
|
||||
from grove.factory import Factory
|
||||
button = Factory.getButton('GPIO-HIGH', 5)
|
||||
|
||||
audio = pyaudio.PyAudio()
|
||||
microphone_card_number = 1
|
||||
speaker_card_number = 1
|
||||
rate = 48000
|
||||
|
||||
def capture_audio():
|
||||
stream = audio.open(format = pyaudio.paInt16,
|
||||
rate = rate,
|
||||
channels = 1,
|
||||
input_device_index = microphone_card_number,
|
||||
input = True,
|
||||
frames_per_buffer = 4096)
|
||||
|
||||
frames = []
|
||||
|
||||
while button.is_pressed():
|
||||
frames.append(stream.read(4096))
|
||||
|
||||
stream.stop_stream()
|
||||
stream.close()
|
||||
|
||||
wav_buffer = io.BytesIO()
|
||||
with wave.open(wav_buffer, 'wb') as wavefile:
|
||||
wavefile.setnchannels(1)
|
||||
wavefile.setsampwidth(audio.get_sample_size(pyaudio.paInt16))
|
||||
wavefile.setframerate(rate)
|
||||
wavefile.writeframes(b''.join(frames))
|
||||
wav_buffer.seek(0)
|
||||
|
||||
return wav_buffer
|
||||
|
||||
api_key = '<key>'
|
||||
location = '<location>'
|
||||
language = '<language>'
|
||||
|
||||
def get_access_token():
|
||||
headers = {
|
||||
'Ocp-Apim-Subscription-Key': api_key
|
||||
}
|
||||
|
||||
token_endpoint = f'https://{location}.api.cognitive.microsoft.com/sts/v1.0/issuetoken'
|
||||
response = requests.post(token_endpoint, headers=headers)
|
||||
return str(response.text)
|
||||
|
||||
def convert_speech_to_text(buffer):
|
||||
url = f'https://{location}.stt.speech.microsoft.com/speech/recognition/conversation/cognitiveservices/v1'
|
||||
|
||||
headers = {
|
||||
'Authorization': 'Bearer ' + get_access_token(),
|
||||
'Content-Type': f'audio/wav; codecs=audio/pcm; samplerate={rate}',
|
||||
'Accept': 'application/json;text/xml'
|
||||
}
|
||||
|
||||
params = {
|
||||
'language': language
|
||||
}
|
||||
|
||||
response = requests.post(url, headers=headers, params=params, data=buffer)
|
||||
response_json = json.loads(response.text)
|
||||
|
||||
if response_json['RecognitionStatus'] == 'Success':
|
||||
return response_json['DisplayText']
|
||||
else:
|
||||
return ''
|
||||
|
||||
while True:
|
||||
while not button.is_pressed():
|
||||
time.sleep(.1)
|
||||
|
||||
buffer = capture_audio()
|
||||
text = convert_speech_to_text(buffer)
|
||||
print(text)
|
@ -0,0 +1,22 @@
|
||||
import time
|
||||
from azure.cognitiveservices.speech import SpeechConfig, SpeechRecognizer
|
||||
|
||||
api_key = '<key>'
|
||||
location = '<location>'
|
||||
language = '<language>'
|
||||
|
||||
speech_config = SpeechConfig(subscription=api_key,
|
||||
region=location,
|
||||
speech_recognition_language=language)
|
||||
|
||||
recognizer = SpeechRecognizer(speech_config=speech_config)
|
||||
|
||||
def recognized(args):
|
||||
print(args.result.text)
|
||||
|
||||
recognizer.recognized.connect(recognized)
|
||||
|
||||
recognizer.start_continuous_recognition()
|
||||
|
||||
while True:
|
||||
time.sleep(1)
|
@ -0,0 +1,213 @@
|
||||
# Capture audio - Raspberry Pi
|
||||
|
||||
In this part of the lesson, you will write code to capture audio on your Raspberry Pi. Audio capture will be controlled by a button.
|
||||
|
||||
## Hardware
|
||||
|
||||
The Raspberry Pi needs a button to control the audio capture.
|
||||
|
||||
The button you will use is a Grove button. This is a digital sensor that turns a signal on or off. These buttons can be configured to send a high signal when the button is pressed, and low when it is not, or low when pressed and high when not.
|
||||
|
||||
If you are using a ReSpeaker 2-Mics Pi HAT as a microphone, then there is no need to connect a button as this hat has one fitted already. Skip to the next section.
|
||||
|
||||
### Connect the button
|
||||
|
||||
The button can be connected to the Grove base hat.
|
||||
|
||||
#### Task - connect the button
|
||||
|
||||

|
||||
|
||||
1. Insert one end of a Grove cable into the socket on the button module. It will only go in one way round.
|
||||
|
||||
1. With the Raspberry Pi powered off, connect the other end of the Grove cable to the digital socket marked **D5** on the Grove Base hat attached to the Pi. This socket is the second from the left, on the row of sockets next to the GPIO pins.
|
||||
|
||||

|
||||
|
||||
## Capture audio
|
||||
|
||||
You can capture audio from the microphone using Python code.
|
||||
|
||||
### Task - capture audio
|
||||
|
||||
1. Power up the Pi and wait for it to boot
|
||||
|
||||
1. Launch VS Code, either directly on the Pi, or connect via the Remote SSH extension.
|
||||
|
||||
1. The PyAudio Pip package has functions to record and play back audio. This package depends on some audio libraries that need to be installed first. Run the following commands in the terminal to install these:
|
||||
|
||||
```sh
|
||||
sudo apt update
|
||||
sudo apt install libportaudio0 libportaudio2 libportaudiocpp0 portaudio19-dev libasound2-plugins --yes
|
||||
```
|
||||
|
||||
1. Install the PyAudio Pip package.
|
||||
|
||||
```sh
|
||||
pip3 install pyaudio
|
||||
```
|
||||
|
||||
1. Create a new folder called `smart-timer` and add a file called `app.py` to this folder.
|
||||
|
||||
1. Add the following imports to the top of this file:
|
||||
|
||||
```python
|
||||
import io
|
||||
import pyaudio
|
||||
import time
|
||||
import wave
|
||||
|
||||
from grove.factory import Factory
|
||||
```
|
||||
|
||||
This imports the `pyaudio` module, some standard Python modules to handle wave files, and the `grove.factory` module to import a `Factory` to create a button class.
|
||||
|
||||
1. Below this, add code to create a Grove button.
|
||||
|
||||
If you are using the ReSpeaker 2-Mics Pi HAT, use the following code:
|
||||
|
||||
```python
|
||||
# The button on the ReSpeaker 2-Mics Pi HAT
|
||||
button = Factory.getButton("GPIO-LOW", 17)
|
||||
```
|
||||
|
||||
This creates a button on port **D17**, the port that the button on the ReSpeaker 2-Mics Pi HAT is connected to. This button is set to send a low signal when pressed.
|
||||
|
||||
If you are not using the ReSpeaker 2-Mics Pi HAT, and are using a Grove button connected to the base hat, use this code.
|
||||
|
||||
```python
|
||||
button = Factory.getButton("GPIO-HIGH", 5)
|
||||
```
|
||||
|
||||
This creates a button on port **D5** that is set to send a high signal when pressed.
|
||||
|
||||
1. Below this, create an instance of the PyAudio class to handle audio:
|
||||
|
||||
```python
|
||||
audio = pyaudio.PyAudio()
|
||||
```
|
||||
|
||||
1. Declare the hardware card number for the microphone and speaker. This will be the number of the card you found by running `arecord -l` and `aplay -l` earlier in this lesson.
|
||||
|
||||
```python
|
||||
microphone_card_number = <microphone card number>
|
||||
speaker_card_number = <speaker card number>
|
||||
```
|
||||
|
||||
Replace `<microphone card number>` with the number of your microphones card.
|
||||
|
||||
Replace `<speaker card number>` with the number of your speakers card, the same number you set in the `alsa.conf` file.
|
||||
|
||||
1. Below this, declare the sample rate to use for the audio capture and playback. You may need to change this depending on the hardware you are using.
|
||||
|
||||
```python
|
||||
rate = 48000 #48KHz
|
||||
```
|
||||
|
||||
If you get sample rate errors when running this code later, change this value to `44100` or `16000`. The higher the value, the better the quality of the sound.
|
||||
|
||||
1. Below this, create a new function called `capture_audio`. This will be called to capture audio from the microphone:
|
||||
|
||||
```python
|
||||
def capture_audio():
|
||||
```
|
||||
|
||||
1. Inside this function, add the following to capture the audio:
|
||||
|
||||
```python
|
||||
stream = audio.open(format = pyaudio.paInt16,
|
||||
rate = rate,
|
||||
channels = 1,
|
||||
input_device_index = microphone_card_number,
|
||||
input = True,
|
||||
frames_per_buffer = 4096)
|
||||
|
||||
frames = []
|
||||
|
||||
while button.is_pressed():
|
||||
frames.append(stream.read(4096))
|
||||
|
||||
stream.stop_stream()
|
||||
stream.close()
|
||||
```
|
||||
|
||||
This code opens an audio input stream using the PyAudio object. This stream will capture audio from the microphone at 16KHz, capturing it in buffers of 4096 bytes in size.
|
||||
|
||||
The code then loops whilst the Grove button is pressed, reading these 4096 byte buffers into an array each time.
|
||||
|
||||
> 💁 You can read more on the options passed to the `open` method in the [PyAudio documentation](https://people.csail.mit.edu/hubert/pyaudio/docs/).
|
||||
|
||||
Once the button is released, the stream is stopped and closed.
|
||||
|
||||
1. Add the following to the end of this function:
|
||||
|
||||
```python
|
||||
wav_buffer = io.BytesIO()
|
||||
with wave.open(wav_buffer, 'wb') as wavefile:
|
||||
wavefile.setnchannels(1)
|
||||
wavefile.setsampwidth(audio.get_sample_size(pyaudio.paInt16))
|
||||
wavefile.setframerate(rate)
|
||||
wavefile.writeframes(b''.join(frames))
|
||||
wav_buffer.seek(0)
|
||||
|
||||
return wav_buffer
|
||||
```
|
||||
|
||||
This code creates a binary buffer, and writes all the captured audio to it as a [WAV file](https://wikipedia.org/wiki/WAV). This is a standard way to write uncompressed audio to a file. This buffer is then returned.
|
||||
|
||||
1. Add the following `play_audio` function to play back the audio buffer:
|
||||
|
||||
```python
|
||||
def play_audio(buffer):
|
||||
stream = audio.open(format = pyaudio.paInt16,
|
||||
rate = rate,
|
||||
channels = 1,
|
||||
output_device_index = speaker_card_number,
|
||||
output = True)
|
||||
|
||||
with wave.open(buffer, 'rb') as wf:
|
||||
data = wf.readframes(4096)
|
||||
|
||||
while len(data) > 0:
|
||||
stream.write(data)
|
||||
data = wf.readframes(4096)
|
||||
|
||||
stream.close()
|
||||
```
|
||||
|
||||
This function opens another audio stream, this time for output - to play the audio. It uses the same settings as the input stream. The buffer is then opened as a wave file and written to the output stream in 4096 byte chunks, playing the audio. The stream is then closed.
|
||||
|
||||
1. Add the following code below the `capture_audio` function to loop until the button is pressed. Once the button is pressed, the audio is captured, then played.
|
||||
|
||||
```python
|
||||
while True:
|
||||
while not button.is_pressed():
|
||||
time.sleep(.1)
|
||||
|
||||
buffer = capture_audio()
|
||||
play_audio(buffer)
|
||||
```
|
||||
|
||||
1. Run the code. Press the button and speak into the microphone. Release the button when you are done, and you will hear the recording.
|
||||
|
||||
You may get some ALSA errors when the PyAudio instance is created. This is due to configuration on the Pi for audio devices you don't have. You can ignore these errors.
|
||||
|
||||
```output
|
||||
pi@raspberrypi:~/smart-timer $ python3 app.py
|
||||
ALSA lib pcm.c:2565:(snd_pcm_open_noupdate) Unknown PCM cards.pcm.front
|
||||
ALSA lib pcm.c:2565:(snd_pcm_open_noupdate) Unknown PCM cards.pcm.rear
|
||||
ALSA lib pcm.c:2565:(snd_pcm_open_noupdate) Unknown PCM cards.pcm.center_lfe
|
||||
ALSA lib pcm.c:2565:(snd_pcm_open_noupdate) Unknown PCM cards.pcm.side
|
||||
```
|
||||
|
||||
If you get the following error:
|
||||
|
||||
```output
|
||||
OSError: [Errno -9997] Invalid sample rate
|
||||
```
|
||||
|
||||
then change the `rate` to either 44100 or 16000.
|
||||
|
||||
> 💁 You can find this code in the [code-record/pi](code-record/pi) folder.
|
||||
|
||||
😀 Your audio recording program was a success!
|
@ -0,0 +1,143 @@
|
||||
# Configure your microphone and speakers - Raspberry Pi
|
||||
|
||||
In this part of the lesson, you will add a microphone and speakers to your Raspberry Pi.
|
||||
|
||||
## Hardware
|
||||
|
||||
The Raspberry Pi needs a microphone.
|
||||
|
||||
The Pi doesn't have a microphone built in, you will need to add an external microphone. There are multiple ways to do this:
|
||||
|
||||
* USB microphone
|
||||
* USB headset
|
||||
* USB all in one speakerphone
|
||||
* USB audio adapter and microphone with a 3.5mm jack
|
||||
* [ReSpeaker 2-Mics Pi HAT](https://www.seeedstudio.com/ReSpeaker-2-Mics-Pi-HAT.html)
|
||||
|
||||
> 💁 Bluetooth microphones are not all supported on the Raspberry Pi, so if you have a bluetooth microphone or headset, you may have issues pairing or capturing audio.
|
||||
|
||||
Raspberry Pis come with a 3.5mm headphone jack. You can use this to connect headphones, a headset or a speaker. You can also add speakers using:
|
||||
|
||||
* HDMI audio through a monitor or TV
|
||||
* USB speakers
|
||||
* USB headset
|
||||
* USB all in one speakerphone
|
||||
* [ReSpeaker 2-Mics Pi HAT](https://www.seeedstudio.com/ReSpeaker-2-Mics-Pi-HAT.html) with a speaker attached, either to the 3.5mm jack or to the JST port
|
||||
|
||||
## Connect and configure the microphone and speakers
|
||||
|
||||
The microphone and speakers need to be connected, and configured.
|
||||
|
||||
### Task - connect and configure the microphone
|
||||
|
||||
1. Connect the microphone using the appropriate method. For example, connect it via one of the USB ports.
|
||||
|
||||
1. If you are using the ReSpeaker 2-Mics Pi HAT, you can remove the Grove base hat, then fit the ReSpeaker hat in it's place.
|
||||
|
||||

|
||||
|
||||
You will need a Grove button later in this lesson, but one is built into this hat, so the Grove base hat is not needed.
|
||||
|
||||
Once the hat is fitted, you will need to install some drivers. Refer to the [Seeed getting started instructions](https://wiki.seeedstudio.com/ReSpeaker_2_Mics_Pi_HAT_Raspberry/#getting-started) for driver installation instructions.
|
||||
|
||||
> ⚠️ The instructions use `git` to clone a repository. If you don't have `git` installed on your Pi, you can install it by running the following command:
|
||||
>
|
||||
> ```sh
|
||||
> sudo apt install git --yes
|
||||
> ```
|
||||
|
||||
1. Run the following command in your Terminal either on the Pi, or connected using VS Code and a remote SSH session to see information about the connected microphone:
|
||||
|
||||
```sh
|
||||
arecord -l
|
||||
```
|
||||
|
||||
You will see a list of connected microphones. It will be something like the following:
|
||||
|
||||
```output
|
||||
pi@raspberrypi:~ $ arecord -l
|
||||
**** List of CAPTURE Hardware Devices ****
|
||||
card 1: M0 [eMeet M0], device 0: USB Audio [USB Audio]
|
||||
Subdevices: 1/1
|
||||
Subdevice #0: subdevice #0
|
||||
```
|
||||
|
||||
Assuming you only have one microphone, you should only see one entry. Configuration of mics can be tricky on Linux, so it is easiest to only use one microphone and unplug any others.
|
||||
|
||||
Note down the card number, as you will need this later. In the output above the card number is 1.
|
||||
|
||||
### Task - connect and configure the speaker
|
||||
|
||||
1. Connect the speakers using the appropriate method.
|
||||
|
||||
1. Run the following command in your Terminal either on the Pi, or connected using VS Code and a remote SSH session to see information about the connected speakers:
|
||||
|
||||
```sh
|
||||
aplay -l
|
||||
```
|
||||
|
||||
You will see a list of connected speakers. It will be something like the following:
|
||||
|
||||
```output
|
||||
pi@raspberrypi:~ $ aplay -l
|
||||
**** List of PLAYBACK Hardware Devices ****
|
||||
card 0: Headphones [bcm2835 Headphones], device 0: bcm2835 Headphones [bcm2835 Headphones]
|
||||
Subdevices: 8/8
|
||||
Subdevice #0: subdevice #0
|
||||
Subdevice #1: subdevice #1
|
||||
Subdevice #2: subdevice #2
|
||||
Subdevice #3: subdevice #3
|
||||
Subdevice #4: subdevice #4
|
||||
Subdevice #5: subdevice #5
|
||||
Subdevice #6: subdevice #6
|
||||
Subdevice #7: subdevice #7
|
||||
card 1: M0 [eMeet M0], device 0: USB Audio [USB Audio]
|
||||
Subdevices: 1/1
|
||||
Subdevice #0: subdevice #0
|
||||
```
|
||||
|
||||
You will always see `card 0: Headphones` as this is the built-in headphone jack. If you have added additional speakers, such as a USB speaker, you will see this listed as well.
|
||||
|
||||
1. If you are using an additional speaker, and not a speaker or headphones connected to the built-in headphone jack, you need to configure it as the default. To do this run the following command:
|
||||
|
||||
```sh
|
||||
sudo nano /usr/share/alsa/alsa.conf
|
||||
```
|
||||
|
||||
This will open a configuration file in `nano`, a terminal-based text editor. Scroll down using the arrow keys on your keyboard until you find the following line:
|
||||
|
||||
```output
|
||||
defaults.pcm.card 0
|
||||
```
|
||||
|
||||
Change the value from `0` to the card number of the card you want to use from the list that came back from the call to `aplay -l`. For example, in the output above there is a second sound card called `card 1: M0 [eMeet M0], device 0: USB Audio [USB Audio]`, using card 1. To use this, I would update the line to be:
|
||||
|
||||
```output
|
||||
defaults.pcm.card 1
|
||||
```
|
||||
|
||||
Set this value to the appropriate card number. You can navigate to the number using the arrow keys on your keyboard, then delete and type the new number as normal when editing text files.
|
||||
|
||||
1. Save the changes and close the file by pressing `Ctrl+x`. Press `y` to save the file, then `return` to select the file name.
|
||||
|
||||
### Task - test the microphone and speaker
|
||||
|
||||
1. Run the following command to record 5 seconds of audio through the microphone:
|
||||
|
||||
```sh
|
||||
arecord --format=S16_LE --duration=5 --rate=16000 --file-type=wav out.wav
|
||||
```
|
||||
|
||||
Whilst this command is running, make noise into the microphone such as by speaking, singing, beat boxing, playing an instrument or whatever takes your fancy.
|
||||
|
||||
1. After 5 seconds, the recording will stop. Run the following command to play back the audio:
|
||||
|
||||
```sh
|
||||
aplay --format=S16_LE --rate=16000 out.wav
|
||||
```
|
||||
|
||||
You will hear the audio bing played back through the speakers. Adjust the output volume on your speaker as necessary.
|
||||
|
||||
1. If you need to adjust the volume of the built-in microphone port, or adjust the gain of the microphone, you can use the `alsamixer` utility. You can read more on this utility on thw [Linux alsamixer man page](https://linux.die.net/man/1/alsamixer)
|
||||
|
||||
1. If you get errors playing back the audio, check the card you set as the `defaults.pcm.card` in the `alsa.conf` file.
|
@ -0,0 +1,106 @@
|
||||
# Speech to text - Raspberry Pi
|
||||
|
||||
In this part of the lesson, you will write code to convert speech in the captured audio to text using the speech service.
|
||||
|
||||
## Send the audio to the speech service
|
||||
|
||||
The audio can be sent to the speech service using the REST API. To use the speech service, first you need to request an access token, then use that token to access the REST API. These access tokens expire after 10 minutes, so your code should request them on a regular basis to ensure they are always up to date.
|
||||
|
||||
### Task - get an access token
|
||||
|
||||
1. Open the `smart-timer` project on your Pi.
|
||||
|
||||
1. Remove the `play_audio` function. This is no longer needed as you don't want a smart timer to repeat back to you what you said.
|
||||
|
||||
1. Add the following imports to the top of the `app.py` file:
|
||||
|
||||
```python
|
||||
import requests
|
||||
import json
|
||||
```
|
||||
|
||||
1. Add the following code above the `while True` loop to declare some settings for the speech service:
|
||||
|
||||
```python
|
||||
api_key = '<key>'
|
||||
location = '<location>'
|
||||
language = '<language>'
|
||||
```
|
||||
|
||||
Replace `<key>` with the API key for your speech service. Replace `<location>` with the location you used when you created the speech service resource.
|
||||
|
||||
Replace `<language>` with the locale name for language you will be speaking in, for example `en-GB` for English, or `zn-HK` for Cantonese. You can find a list of the supported languages and their locale names in the [Language and voice support documentation on Microsoft docs](https://docs.microsoft.com/azure/cognitive-services/speech-service/language-support?WT.mc_id=academic-17441-jabenn#speech-to-text).
|
||||
|
||||
1. Below this, add the following function to get an access token:
|
||||
|
||||
```python
|
||||
def get_access_token():
|
||||
headers = {
|
||||
'Ocp-Apim-Subscription-Key': api_key
|
||||
}
|
||||
|
||||
token_endpoint = f'https://{location}.api.cognitive.microsoft.com/sts/v1.0/issuetoken'
|
||||
response = requests.post(token_endpoint, headers=headers)
|
||||
return str(response.text)
|
||||
```
|
||||
|
||||
This calls a token issuing endpoint, passing the API key as a header. This call returns an access token that can be used to call the speech services.
|
||||
|
||||
1. Below this, declare a function to convert speech in the captured audio to text using the REST API:
|
||||
|
||||
```python
|
||||
def convert_speech_to_text(buffer):
|
||||
```
|
||||
|
||||
1. Inside this function, set up the REST API URL and headers:
|
||||
|
||||
```python
|
||||
url = f'https://{location}.stt.speech.microsoft.com/speech/recognition/conversation/cognitiveservices/v1'
|
||||
|
||||
headers = {
|
||||
'Authorization': 'Bearer ' + get_access_token(),
|
||||
'Content-Type': f'audio/wav; codecs=audio/pcm; samplerate={rate}',
|
||||
'Accept': 'application/json;text/xml'
|
||||
}
|
||||
|
||||
params = {
|
||||
'language': language
|
||||
}
|
||||
```
|
||||
|
||||
This builds a URL using the location of the speech services resource. It then populates the headers with the access token from the `get_access_token` function, as well as the sample rate used to capture the audio. Finally it defines some parameters to be passed with the URL containing the language in the audio.
|
||||
|
||||
1. Below this, add the following code to call the REST API and get back the text:
|
||||
|
||||
```python
|
||||
response = requests.post(url, headers=headers, params=params, data=buffer)
|
||||
response_json = json.loads(response.text)
|
||||
|
||||
if response_json['RecognitionStatus'] == 'Success':
|
||||
return response_json['DisplayText']
|
||||
else:
|
||||
return ''
|
||||
```
|
||||
|
||||
This calls the URL and decodes the JSON value that comes in the response. The `RecognitionStatus` value in the response indicates if the call was able to extract speech into text successfully, and if this is `Success` then the text is returned from the function, otherwise an empty string is returned.
|
||||
|
||||
1. Finally replace the call to `play_audio` in the `while True` loop with a call to the `convert_speech_to_text` function, as well as printing the text to the console:
|
||||
|
||||
```python
|
||||
text = convert_speech_to_text(buffer)
|
||||
print(text)
|
||||
```
|
||||
|
||||
1. Run the code. Press the button and speak into the microphone. Release the button when you are done, and the audio will be converted to text and printed to the console.
|
||||
|
||||
```output
|
||||
pi@raspberrypi:~/smart-timer $ python3 app.py
|
||||
Hello world.
|
||||
Welcome to IoT for beginners.
|
||||
```
|
||||
|
||||
Try different types of sentences, along with sentences where words sound the same but have different meanings. For example, if you are speaking in English, say 'I want to buy two bananas and an apple too', and notice how it will use the correct to, two and too based on the context of the word, not just it's sound.
|
||||
|
||||
> 💁 You can find this code in the [code-speech-to-text/pi](code-speech-to-text/pi) folder.
|
||||
|
||||
😀 Your speech to text program was a success!
|
@ -0,0 +1,3 @@
|
||||
# Capture audio - Virtual IoT device
|
||||
|
||||
The Python libraries that you will be using later in this lesson to convert speech to text have built-in audio capture on Windows, macOS and Linux. You don't need to do anything here.
|
@ -0,0 +1,12 @@
|
||||
# Configure your microphone and speakers - Virtual IoT Hardware
|
||||
|
||||
The virtual IoT hardware will use a microphone and speakers attached to your computer.
|
||||
|
||||
If your computer doesn't have a microphone and speakers built in, you will need to attach these using hardware of your choice, such as:
|
||||
|
||||
* USB microphone
|
||||
* USB speakers
|
||||
* Speakers built into your monitor and connected over HDMI
|
||||
* Bluetooth headset
|
||||
|
||||
Refer to your hardware manufacturers instructions to install and configure this hardware.
|
@ -0,0 +1,95 @@
|
||||
# Speech to text - Virtual IoT device
|
||||
|
||||
In this part of the lesson, you will write code to convert speech captured from your microphone to text using the speech service.
|
||||
|
||||
## Convert speech to text
|
||||
|
||||
On Windows, Linux, and macOS, the speech services Python SDK can be used to listen to your microphone and convert any speech that is detected to text. It will listen continuously, detecting the audio levels and sending the speech for conversion to text when the audio level drops, such as at the end of a block of speech.
|
||||
|
||||
### Task - convert speech to text
|
||||
|
||||
1. Create a new Python app on your computer in a folder called `smart-timer` with a single file called `app.py` and a Python virtual environment.
|
||||
|
||||
1. Install the Pip package for the speech services. Make sure you are installing this from a terminal with the virtual environment activated.
|
||||
|
||||
```sh
|
||||
pip install azure-cognitiveservices-speech
|
||||
```
|
||||
|
||||
> ⚠️ If you get the following error:
|
||||
>
|
||||
> ```output
|
||||
> ERROR: Could not find a version that satisfies the requirement azure-cognitiveservices-speech (from versions: none)
|
||||
> ERROR: No matching distribution found for azure-cognitiveservices-speech
|
||||
> ```
|
||||
>
|
||||
> You will need to update Pip. Do this with the following command, then try to install the package again
|
||||
>
|
||||
> ```sh
|
||||
> pip install --upgrade pip
|
||||
> ```
|
||||
|
||||
1. Add the following imports to the `app,py` file:
|
||||
|
||||
```python
|
||||
import time
|
||||
from azure.cognitiveservices.speech import SpeechConfig, SpeechRecognizer
|
||||
```
|
||||
|
||||
This imports some classes used to recognize speech.
|
||||
|
||||
1. Add the following code to declare some configuration:
|
||||
|
||||
```python
|
||||
api_key = '<key>'
|
||||
location = '<location>'
|
||||
language = '<language>'
|
||||
|
||||
speech_config = SpeechConfig(subscription=api_key,
|
||||
region=location,
|
||||
speech_recognition_language=language)
|
||||
```
|
||||
|
||||
Replace `<key>` with the API key for your speech service. Replace `<location>` with the location you used when you created the speech service resource.
|
||||
|
||||
Replace `<language>` with the locale name for language you will be speaking in, for example `en-GB` for English, or `zn-HK` for Cantonese. You can find a list of the supported languages and their locale names in the [Language and voice support documentation on Microsoft docs](https://docs.microsoft.com/azure/cognitive-services/speech-service/language-support?WT.mc_id=academic-17441-jabenn#speech-to-text).
|
||||
|
||||
This configuration is then used to create a `SpeechConfig` object that will be used to configure the speech services.
|
||||
|
||||
1. Add the following code to create a speech recognizer:
|
||||
|
||||
```python
|
||||
recognizer = SpeechRecognizer(speech_config=speech_config)
|
||||
```
|
||||
|
||||
1. The speech recognizer runs on a background thread, listening for audio and converting any speech in it to text. You can get the text using a callback function - a function you define and pass to the recognizer. Every time speech is detected, the callback is called. Add the following code to define a callback that prints the text to the console, and pass this callback to the recognizer:
|
||||
|
||||
```python
|
||||
def recognized(args):
|
||||
print(args.result.text)
|
||||
|
||||
recognizer.recognized.connect(recognized)
|
||||
```
|
||||
|
||||
1. The recognizer only starts listening when you explicitly start it. Add the following code to start the recognition. This runs in the background, so your application will also need an infinite loop that sleeps to keep the application running.
|
||||
|
||||
```python
|
||||
recognizer.start_continuous_recognition()
|
||||
|
||||
while True:
|
||||
time.sleep(1)
|
||||
```
|
||||
|
||||
1. Run this app. Speak into your microphone and the audio converted to text will be output to the console.
|
||||
|
||||
```output
|
||||
(.venv) ➜ smart-timer python3 app.py
|
||||
Hello world.
|
||||
Welcome to IoT for beginners.
|
||||
```
|
||||
|
||||
Try different types of sentences, along with sentences where words sound the same but have different meanings. For example, if you are speaking in English, say 'I want to buy two bananas and an apple too', and notice how it will use the correct to, two and too based on the context of the word, not just it's sound.
|
||||
|
||||
> 💁 You can find this code in the [code-speech-to-text/virtual-iot-device](code-speech-to-text/virtual-iot-device) folder.
|
||||
|
||||
😀 Your speech to text program was a success!
|
@ -0,0 +1,3 @@
|
||||
# Capture audio - Wio Terminal
|
||||
|
||||
Coming soon!
|
@ -0,0 +1,3 @@
|
||||
# Configure your microphone and speakers - Wio Terminal
|
||||
|
||||
Coming soon!
|
@ -0,0 +1,3 @@
|
||||
# Speech to text - Wio Terminal
|
||||
|
||||
Coming soon!
|
@ -0,0 +1,431 @@
|
||||
# Understand language
|
||||
|
||||
Add a sketchnote if possible/appropriate
|
||||
|
||||

|
||||
|
||||
## Pre-lecture quiz
|
||||
|
||||
[Pre-lecture quiz](https://brave-island-0b7c7f50f.azurestaticapps.net/quiz/33)
|
||||
|
||||
## Introduction
|
||||
|
||||
In the last lesson you converted speech to text. For this to be used to program a smart timer, your code will need to have an understanding of what was said. You could assume the user will speak a fixed phrase, such as "Set a 3 minute timer", and parse that expression to get how long the timer should be, but this isn't very user-friendly. If a user were to say "Set a timer for 3 minutes", you or I would understand what they mean, but your code would not, it would be expecting a fixed phrase.
|
||||
|
||||
This is where language understanding comes in, using AI models to interpret text and return the details that are needed, for example being able to take both "Set a 3 minute timer" and "Set a timer for 3 minutes", and understand that a timer is required for 3 minutes.
|
||||
|
||||
In this lesson you will learn about language understanding models, how to create them, train them, and use them from your code.
|
||||
|
||||
In this lesson we'll cover:
|
||||
|
||||
* [Language understanding](#language-understanding)
|
||||
* [Create a language understanding model](create-a-language-understanding-model)
|
||||
* [Intents and entities](#intents-and-entities)
|
||||
* [Use the language understanding model](#use-the-language-understanding-model)
|
||||
|
||||
## Language understanding
|
||||
|
||||
Humans have used language to communicate for hundreds of thousands of years. We communicate with words, sounds, or actions and understand what is said, both the meaning of the words, sounds or actions, but also their context. We understand sincerity and sarcasm, allowing the same words to mean different things depending on the tone of our voice.
|
||||
|
||||
✅ Think about some of the conversations you have had recently. How much of the conversation would be hard for a computer to understand because it needs context?
|
||||
|
||||
Language understanding, also called natural-language understanding is part of a field of artificial intelligence called natural-language processing (or NLP), and deals with reading comprehension, trying to understand the details of words or sentences. If you use a voice assistant such as Alexa or Siri, you have used language understanding services. These are the behind-the-scenes AI services that convert "Alexa, play the latest album by Taylor Swift" into my daughter dancing around the living room to her favorite tunes.
|
||||
|
||||
> 💁 Computers, despite all their advances, still have a long way to go to truly understand text. When we refer to language understanding with computers, we don't mean anything anywhere near as advanced as human communication, instead we mean taking some words and extracting key details.
|
||||
|
||||
As humans, we understand language without really thinking about it. If I asked another human to "play the latest album by Taylor Swift" then they would instinctively know what I meant. For a computer, this is harder. It would have to take the words, converted from speech to text, and work out the following pieces of information:
|
||||
|
||||
* Music needs to be played
|
||||
* The music is by the artist Taylor Swift
|
||||
* The specific music is a whole album of multiple tracks in order
|
||||
* Taylor Swift has many albums, so they need to be sorted by chronological order and the most recently published is the one required
|
||||
|
||||
✅ Think of some other sentences you have spoken when making requests, such as ordering coffee or asking a family member to pass you something. Try to break then down into the pieces of information a computer would need to extract to understand the sentence.
|
||||
|
||||
Language understanding models are AI models that are trained to extract certain details from language, and then are trained for specific tasks using transfer learning, in the same way you trained a Custom Vision model using a small set of images. You can take a model, then train it using the text you want it to understand.
|
||||
|
||||
## Create a language understanding model
|
||||
|
||||

|
||||
|
||||
You can create language understanding models using LUIS, a language understanding service from Microsoft that is part of Cognitive Services.
|
||||
|
||||
### Task - create an authoring resource
|
||||
|
||||
To use LUIS, you need to create an authoring resource.
|
||||
|
||||
1. Use the following command to create an authoring resource in your `smart-timer` resource group:
|
||||
|
||||
```python
|
||||
az cognitiveservices account create --name smart-timer-luis-authoring \
|
||||
--resource-group smart-timer \
|
||||
--kind LUIS.Authoring \
|
||||
--sku F0 \
|
||||
--yes \
|
||||
--location <location>
|
||||
```
|
||||
|
||||
Replace `<location>` with the location you used when creating the Resource Group.
|
||||
|
||||
> ⚠️ LUIS isn't available in all regions, so if you get the following error:
|
||||
>
|
||||
> ```output
|
||||
> InvalidApiSetId: The account type 'LUIS.Authoring' is either invalid or unavailable in given region.
|
||||
> ```
|
||||
>
|
||||
> pick a different region.
|
||||
|
||||
This will create a free-tier LUIS authoring resource.
|
||||
|
||||
### Task - create a language understanding app
|
||||
|
||||
1. Open the LUIS portal at [luis.ai](https://luis.ai?WT.mc_id=academic-17441-jabenn) in your browser, and sign in with the same account you have been using for Azure.
|
||||
|
||||
1. Follow the instructions on the dialog to select your Azure subscription, then select the `smart-timer-luis-authoring` resource you have just created.
|
||||
|
||||
1. From the *Conversation apps* list, select the **New app** button to create a new application. Name the new app `smart-timer`, and set the *Culture* to your language.
|
||||
|
||||
> 💁 There is a field for a prediction resource. You can create a second resource just for prediction, but the free authoring resource allows 1,000 predictions a month which should be enough for development, so you can leave this blank.
|
||||
|
||||
1. Read through the guide that appears once you cerate the app to get an understanding of the steps you need to take to train the language understanding model. Close this guide when you are done.
|
||||
|
||||
## Intents and entities
|
||||
|
||||
Language understanding is based around *intents* and *entities*. Intents are what the intent of the words are, for example playing music, setting a timer, or ordering food. Entities are what the intent is referring to, such as the album, the length of the timer, or the type of food. Each sentence that the model interprets should have at least one intent, and optionally one or more entities.
|
||||
|
||||
Some examples:
|
||||
|
||||
| Sentence | Intent | Entities |
|
||||
| --------------------------------------------------- | ---------------- | ------------------------------------------ |
|
||||
| "Play the latest album by Taylor Swift" | *play music* | *the latest album by Taylor Swift* |
|
||||
| "Set a 3 minute timer" | *set a timer* | *3 minutes* |
|
||||
| "Cancel my timer" | *cancel a timer* | None |
|
||||
| "Order 3 large pineapple pizzas and a caesar salad" | *order food* | *3 large pineapple pizzas*, *caesar salad* |
|
||||
|
||||
✅ With the sentences you though about earlier, what would be the intent and any entities in that sentence?
|
||||
|
||||
To train LUIS, first you set the entities. These can be a fixed list of terms, or learned from the text. For example, you could provide a fixed list of food available from your menu, with variations (or synonyms) of each word, such as *egg plant* and *aubergine* as variations of *aubergine*. LUIS also has pre-built entities that can be used, such as numbers and locations.
|
||||
|
||||
For setting a timer, you could have one entity using the pre-built number entities for the time, and another for the units, such as minutes and seconds. Each unit would have multiple variations to cover the singular and plural forms - such as minute and minutes.
|
||||
|
||||
Once the entities are defined, you create intents. These are learned by the model based on example sentences that you provide (known as utterances). For example, for a *set timer* intent, you might provide the following sentences:
|
||||
|
||||
* `set a 1 second timer`
|
||||
* `set a timer for 1 minute and 12 seconds`
|
||||
* `set a timer for 3 minutes`
|
||||
* `set a 9 minute 30 second timer`
|
||||
|
||||
You then tell LUIS what parts of these sentences map to the entities:
|
||||
|
||||

|
||||
|
||||
The sentence `set a timer for 1 minute and 12 seconds` has the intent of `set timer`. It also has 2 entities with 2 values each:
|
||||
|
||||
| | time | unit |
|
||||
| ---------- | ---: | ------ |
|
||||
| 1 minute | 1 | minute |
|
||||
| 12 seconds | 12 | second |
|
||||
|
||||
To train a good model, you need a range of different example sentences to cover the many different ways someone might ask for the same thing.
|
||||
|
||||
> 💁 As with any AI model, the more data and the more accurate the data you use to train, the better the model.
|
||||
|
||||
✅ Think about the different ways you might ask the same thing and expect a human to understand.
|
||||
|
||||
### Task - add entities to the language understanding models
|
||||
|
||||
For the timer, you need to add 2 entities - one for the unit of time (minutes or seconds), and one for the number of minutes or seconds.
|
||||
|
||||
You can find instructions for using the LUIS portal in the [Quickstart: Build your app in LUIS portal documentation on Microsoft docs](https://docs.microsoft.com/azure/cognitive-services/luis/luis-get-started-create-app?WT.mc_id=academic-17441-jabenn).
|
||||
|
||||
1. From the LUIS portal, select the *Entities* tab and add the *number* prebuilt entity by selecting the **Add prebuilt entity** button, then selecting *number* from the list.
|
||||
|
||||
1. Create a new entity for the time unit using the **Create** button. Name the entity `time unit` and set the type to *List*. Add values for `minute` and `second` to the *Normalized values* list, adding the singular and plural forms to the *synonyms* list. Press `return` after adding each synonym to add it to the list.
|
||||
|
||||
| Normalized value | Synonyms |
|
||||
| ---------------- | --------------- |
|
||||
| minute | minute, minutes |
|
||||
| second | second, seconds |
|
||||
|
||||
### Task - add intents to the language understanding models
|
||||
|
||||
1. From the *Intents* tab, select the **Create** button to create a new intent. Name this intent `set timer`.
|
||||
|
||||
1. In the examples, enter different ways to set a timer using both minutes, seconds and minutes and seconds combined. Examples could be:
|
||||
|
||||
* `set a 1 second timer`
|
||||
* `set a 4 minute timer`
|
||||
* `set a 9 minute 30 second timer`
|
||||
* `set a timer for 1 minute and 12 seconds`
|
||||
* `set a timer for 3 minutes`
|
||||
* `set a timer for 3 minutes and 1 second`
|
||||
* `set a timer for 1 minute1 and 1 second`
|
||||
* `set a timer for 30 seconds`
|
||||
* `set a timer for 1 second`
|
||||
|
||||
1. As you enter each example, LUIS will start detecting entities, and will underline and label any it finds.
|
||||
|
||||

|
||||
|
||||
### Task - train and test the model
|
||||
|
||||
1. Once the entities and intents are configured, you can train the model using the **Train** button on the top menu. Select this button, and the model should train in a few seconds. The button will be greyed out whilst training, and be re-enabled once done.
|
||||
|
||||
1. Select the **Test** button from the top menu to test the language understanding model. Enter text such as `set a timer for 5 minutes and 4 seconds` and press return. The sentence will appear in a box under the text box that you typed it in to, and blow that will be the *top intent*, or the intent that was detected with the highest probability. This should be `set timer`. The intent name will be followed by the probability that the intent detected was the right one.
|
||||
|
||||
1. Select the **Inspect** option to see a breakdown of the results. You will see the top-scoring intent with it's percentage probability, along with lists of the entities detected.
|
||||
|
||||
1. Close the *Test* pane when you are done testing.
|
||||
|
||||
### Task - publish the model
|
||||
|
||||
To use this model from code, you need to publish it. When publishing from LUIS, you can publish to either a staging environment for testing, or a product environment for a full release. In this lesson, a staging environment is fine.
|
||||
|
||||
1. From the LUIS portal, select the **Publish** button from the top menu.
|
||||
|
||||
1. Make sure *Staging slot* is selected, then select **Done**. You will see a notification when the app is published.
|
||||
|
||||
1. You can test this using curl. To build the curl command, you need three values - the endpoint, the application ID (App ID) and an API key. These can be accessed from the **MANAGE** tab that can be selected from the top menu.
|
||||
|
||||
1. From the *Settings* section, copy the App ID
|
||||
|
||||
1. From the *Azure Resources* section, select *Authoring Resource*, and copy the *Primary Key* and *Endpoint URL*
|
||||
|
||||
1. Run the following curl command in your command prompt or terminal:
|
||||
|
||||
```sh
|
||||
curl "<endpoint url>/luis/prediction/v3.0/apps/<app id>/slots/staging/predict" \
|
||||
--request GET \
|
||||
--get \
|
||||
--data "subscription-key=<primary key>" \
|
||||
--data "verbose=false" \
|
||||
--data "show-all-intents=true" \
|
||||
--data-urlencode "query=<sentence>"
|
||||
```
|
||||
|
||||
Replace `<endpoint url>` with the Endpoint URL from the *Azure Resources* section.
|
||||
|
||||
Replace `<app id>` with the App ID from the *Settings* section.
|
||||
|
||||
Replace `<primary key>` with the Primary Key from the *Azure Resources* section.
|
||||
|
||||
Replace `<sentence>` with the sentence you want to test with.
|
||||
|
||||
1. The output of this call will be a JSON document that details the query, the top intent, and a list of entities broken down by type.
|
||||
|
||||
```JSON
|
||||
{
|
||||
"query": "set a timer for 45 minutes and 12 seconds",
|
||||
"prediction": {
|
||||
"topIntent": "set timer",
|
||||
"intents": {
|
||||
"set timer": {
|
||||
"score": 0.97031575
|
||||
},
|
||||
"None": {
|
||||
"score": 0.02205793
|
||||
}
|
||||
},
|
||||
"entities": {
|
||||
"number": [
|
||||
45,
|
||||
12
|
||||
],
|
||||
"time-unit": [
|
||||
[
|
||||
"minute"
|
||||
],
|
||||
[
|
||||
"second"
|
||||
]
|
||||
]
|
||||
}
|
||||
}
|
||||
}
|
||||
```
|
||||
|
||||
The JSON above came from querying with `set a timer for 45 minutes and 12 seconds`:
|
||||
|
||||
* The `set timer` was the top intent with a probability of 97%.
|
||||
* Two *number* entities were detected, `45` and `12`.
|
||||
* Two *time-unit* entities were detected, `minute` and `second`.
|
||||
|
||||
## Use the language understanding model
|
||||
|
||||
Once published, the LUIS model can be called from code. In the last lesson you sent the recognized speech to an IoT Hub, and you can use serverless code to respond to this and understand what was sent.
|
||||
|
||||
### Task - create a serverless functions app
|
||||
|
||||
1. Create an Azure Functions app called `smart-timer-trigger`.
|
||||
|
||||
1. Add an IoT Hub event trigger to this app called `speech-trigger`.
|
||||
|
||||
1. Set the Event Hub compatible endpoint connection string for your IoT Hub in the `local.settings.json` file, and use the key for that entry in the `function.json` file.
|
||||
|
||||
1. Use the Azurite app as a local storage emulator.
|
||||
|
||||
1. Run your functions app and your IoT device to ensure speech is arriving at the IoT Hub.
|
||||
|
||||
```output
|
||||
Python EventHub trigger processed an event: {"speech": "Set a 3 minute timer."}
|
||||
```
|
||||
|
||||
### Task - use the language understanding model
|
||||
|
||||
1. The SDK for LUIS is available via a Pip package. Add the following line to the `requirements.txt` file to add the dependency on this package:
|
||||
|
||||
```sh
|
||||
azure-cognitiveservices-language-luis
|
||||
```
|
||||
|
||||
1. Make sure the VS Code terminal has the virtual environment activated, and run the following command to install the Pip packages:
|
||||
|
||||
```sh
|
||||
pip install -r requirements.txt
|
||||
```
|
||||
|
||||
1. Add new entries to the `local.settings.json` file for your LUIS API Key, Endpoint URL, and App ID from the **MANAGE** tab of the LUIS portal:
|
||||
|
||||
```JSON
|
||||
"LUIS_KEY": "<primary key>",
|
||||
"LUIS_ENDPOINT_URL": "<endpoint url>",
|
||||
"LUIS_APP_ID": "<app id>"
|
||||
```
|
||||
|
||||
Replace `<endpoint url>` with the Endpoint URL from the *Azure Resources* section of the **MANAGE** tab. This will be `https://<location>.api.cognitive.microsoft.com/`.
|
||||
|
||||
Replace `<app id>` with the App ID from the *Settings* section of the **MANAGE** tab.
|
||||
|
||||
Replace `<primary key>` with the Primary Key from the *Azure Resources* section of the **MANAGE** tab.
|
||||
|
||||
1. Add the following imports to the `__init__.py` file:
|
||||
|
||||
```python
|
||||
import json
|
||||
import os
|
||||
from azure.cognitiveservices.language.luis.runtime import LUISRuntimeClient
|
||||
from msrest.authentication import CognitiveServicesCredentials
|
||||
```
|
||||
|
||||
This imports some system libraries, as well as the libraries to interact with LUIS.
|
||||
|
||||
1. In the `main` method, before it loops through all the events, add the following code:
|
||||
|
||||
```python
|
||||
luis_key = os.environ['LUIS_KEY']
|
||||
endpoint_url = os.environ['LUIS_ENDPOINT_URL']
|
||||
app_id = os.environ['LUIS_APP_ID']
|
||||
|
||||
credentials = CognitiveServicesCredentials(luis_key)
|
||||
client = LUISRuntimeClient(endpoint=endpoint_url, credentials=credentials)
|
||||
```
|
||||
|
||||
This loads the values you added to the `local.settings.json` file for your LUIS app, creates a credentials object with your API key, then creates a LUIS client object to interact with your LUIS app.
|
||||
|
||||
1. Predictions are requested from LUIS by sending a prediction request - a JSON document containing the text to predict. Create this with the following code inside the `for event in events` loop:
|
||||
|
||||
```python
|
||||
event_body = json.loads(event.get_body().decode('utf-8'))
|
||||
prediction_request = { 'query' : event_body['speech'] }
|
||||
```
|
||||
|
||||
This code extracts the speech that was sent to the IoT Hub and uses it to build the prediction request.
|
||||
|
||||
1. This request can then be sent to LUIS, using the staging slot that your app was published to:
|
||||
|
||||
```python
|
||||
prediction_response = client.prediction.get_slot_prediction(app_id, 'Staging', prediction_request)
|
||||
```
|
||||
|
||||
1. The prediction response contains the top intent - the intent with the highest prediction score, along with the entities. If the top intent is `set timer`, then the entities can be read to get the time needed for the timer:
|
||||
|
||||
```python
|
||||
if prediction_response.prediction.top_intent == 'set timer':
|
||||
numbers = prediction_response.prediction.entities['number']
|
||||
time_units = prediction_response.prediction.entities['time unit']
|
||||
total_time = 0
|
||||
```
|
||||
|
||||
The `number` entities wil be an array of numbers. For example, if you said *"Set a four minute 17 second timer."*, then the `number` array will contain 2 integers - 4 and 17.
|
||||
|
||||
The `time unit` entities will be an array of arrays of strings, with each time unit as an array of strings inside the array. For example, if you said *"Set a four minute 17 second timer."*, then the `time unit` array will contain 2 arrays with single values each - `['minute']` and `['second']`.
|
||||
|
||||
The JSON version of these entities for *"Set a four minute 17 second timer."* is:
|
||||
|
||||
```json
|
||||
{
|
||||
"number": [4, 17],
|
||||
"time unit": [
|
||||
["minute"],
|
||||
["second"]
|
||||
]
|
||||
}
|
||||
```
|
||||
|
||||
This code also defines a count for the total time for the timer in seconds. This will be populated by the values from the entities.
|
||||
|
||||
1. The entities aren't linked, but we can make some assumptions about them. They will be in the order spoken, so the position in the array can be used to determine which number matches to which time unit. For example:
|
||||
|
||||
* *"Set a 30 second timer"* - this will have one number, `30`, and one time unit, `second` so the single number will match the single time unit.
|
||||
* *"Set a 2 minute and 30 second timer"* - this will have two numbers, `2` and `30`, and two time units, `minute` and `second` so the first number will be for the first time unit (2 minutes), and the second number for the second time unit (30 seconds).
|
||||
|
||||
The following code gets the count of items in the number entities, and uses that to extract the first item from each array, then the second and so on:
|
||||
|
||||
```python
|
||||
for i in range(0, len(numbers)):
|
||||
number = numbers[i]
|
||||
time_unit = time_units[i][0]
|
||||
```
|
||||
|
||||
For *"Set a four minute 17 second timer."*, this will loop twice, giving the following values:
|
||||
|
||||
| loop count | `number` | `time_unit` |
|
||||
| ---------: | -------: | ----------- |
|
||||
| 0 | 4 | minute |
|
||||
| 1 | 17 | second |
|
||||
|
||||
1. Inside this loop, use the number and time unit to calculate the total time for the timer, adding 60 seconds for each minute, and the number of seconds for any seconds.
|
||||
|
||||
```python
|
||||
if time_unit == 'minute':
|
||||
total_time += number * 60
|
||||
else:
|
||||
total_time += number
|
||||
```
|
||||
|
||||
1. Finally, outside this loop through the entities, log the total time for the timer:
|
||||
|
||||
```python
|
||||
logging.info(f'Timer required for {total_time} seconds')
|
||||
```
|
||||
|
||||
1. Run the function app and speak into your IoT device. You will see the total time for the timer in the function app output:
|
||||
|
||||
```output
|
||||
[2021-06-16T01:38:33.316Z] Executing 'Functions.speech-trigger' (Reason='(null)', Id=39720c37-b9f1-47a9-b213-3650b4d0b034)
|
||||
[2021-06-16T01:38:33.329Z] Trigger Details: PartionId: 0, Offset: 3144-3144, EnqueueTimeUtc: 2021-06-16T01:38:32.7970000Z-2021-06-16T01:38:32.7970000Z, SequenceNumber: 8-8, Count: 1
|
||||
[2021-06-16T01:38:33.605Z] Python EventHub trigger processed an event: {"speech": "Set a four minute 17 second timer."}
|
||||
[2021-06-16T01:38:35.076Z] Timer required for 257 seconds
|
||||
[2021-06-16T01:38:35.128Z] Executed 'Functions.speech-trigger' (Succeeded, Id=39720c37-b9f1-47a9-b213-3650b4d0b034, Duration=1894ms)
|
||||
```
|
||||
|
||||
> 💁 You can find this code in the [code/functions](code/functions) folder.
|
||||
|
||||
---
|
||||
|
||||
## 🚀 Challenge
|
||||
|
||||
There are many ways to request the same thing, such as setting a timer. Think of different ways to do this, and use them as examples in your LUIS app. Test these out, to see how well your model can cope with multiple ways to request a timer.
|
||||
|
||||
## Post-lecture quiz
|
||||
|
||||
[Post-lecture quiz](https://brave-island-0b7c7f50f.azurestaticapps.net/quiz/34)
|
||||
|
||||
## Review & Self Study
|
||||
|
||||
* Read more about LUIS and it's capabilities on the [Language Understanding (LUIS) documentation page on Microsoft docs](https://docs.microsoft.com/azure/cognitive-services/luis/?WT.mc_id=academic-17441-jabenn)
|
||||
* Read more about language understanding on the [Natural-language understanding page on Wikipedia](https://wikipedia.org/wiki/Natural-language_understanding)
|
||||
|
||||
## Assignment
|
||||
|
||||
[Cancel the timer](assignment.md)
|
@ -0,0 +1,14 @@
|
||||
# Cancel the timer
|
||||
|
||||
## Instructions
|
||||
|
||||
So far in this lesson you have trained a model to understand setting a timer. Another useful feature is cancelling a timer - maybe your bread is ready and can be taken out of the oven.
|
||||
|
||||
Add a new intent to your LUIS app to cancel the timer. It won't need any entities, but will need some example sentences. Handle this in your serverless code if it is the top intent, logging that the intent was recognized.
|
||||
|
||||
## Rubric
|
||||
|
||||
| Criteria | Exemplary | Adequate | Needs Improvement |
|
||||
| -------- | --------- | -------- | ----------------- |
|
||||
| Add the cancel timer intent to the LUIS app | Was able to add the intent and train the model | Was able to add the intent but not train the model | Was unable to add the intent and train the model |
|
||||
| Handle the intent in the serverless app | Was able to detect the intent as the top intent and log it | Was able to detect the intent as the top intent | Was unable to detect the intent as the top intent |
|
@ -0,0 +1,15 @@
|
||||
{
|
||||
"version": "2.0",
|
||||
"logging": {
|
||||
"applicationInsights": {
|
||||
"samplingSettings": {
|
||||
"isEnabled": true,
|
||||
"excludedTypes": "Request"
|
||||
}
|
||||
}
|
||||
},
|
||||
"extensionBundle": {
|
||||
"id": "Microsoft.Azure.Functions.ExtensionBundle",
|
||||
"version": "[2.*, 3.0.0)"
|
||||
}
|
||||
}
|
@ -0,0 +1,11 @@
|
||||
{
|
||||
"IsEncrypted": false,
|
||||
"Values": {
|
||||
"FUNCTIONS_WORKER_RUNTIME": "python",
|
||||
"AzureWebJobsStorage": "UseDevelopmentStorage=true",
|
||||
"IOT_HUB_CONNECTION_STRING": "<connection string>",
|
||||
"LUIS_KEY": "<primary key>",
|
||||
"LUIS_ENDPOINT_URL": "<endpoint url>",
|
||||
"LUIS_APP_ID": "<app id>"
|
||||
}
|
||||
}
|
@ -0,0 +1,4 @@
|
||||
# Do not include azure-functions-worker as it may conflict with the Azure Functions platform
|
||||
|
||||
azure-functions
|
||||
azure-cognitiveservices-language-luis
|
@ -0,0 +1,43 @@
|
||||
from typing import List
|
||||
import logging
|
||||
|
||||
import azure.functions as func
|
||||
|
||||
import json
|
||||
import os
|
||||
from azure.cognitiveservices.language.luis.runtime import LUISRuntimeClient
|
||||
from msrest.authentication import CognitiveServicesCredentials
|
||||
|
||||
def main(events: List[func.EventHubEvent]):
|
||||
luis_key = os.environ['LUIS_KEY']
|
||||
endpoint_url = os.environ['LUIS_ENDPOINT_URL']
|
||||
app_id = os.environ['LUIS_APP_ID']
|
||||
|
||||
credentials = CognitiveServicesCredentials(luis_key)
|
||||
client = LUISRuntimeClient(endpoint=endpoint_url, credentials=credentials)
|
||||
|
||||
for event in events:
|
||||
logging.info('Python EventHub trigger processed an event: %s',
|
||||
event.get_body().decode('utf-8'))
|
||||
|
||||
event_body = json.loads(event.get_body().decode('utf-8'))
|
||||
prediction_request = { 'query' : event_body['speech'] }
|
||||
|
||||
prediction_response = client.prediction.get_slot_prediction(app_id, 'Staging', prediction_request)
|
||||
|
||||
if prediction_response.prediction.top_intent == 'set timer':
|
||||
numbers = prediction_response.prediction.entities['number']
|
||||
time_units = prediction_response.prediction.entities['time unit']
|
||||
total_time = 0
|
||||
|
||||
for i in range(0, len(numbers)):
|
||||
number = numbers[i]
|
||||
time_unit = time_units[i][0]
|
||||
|
||||
if time_unit == 'minute':
|
||||
total_time += number * 60
|
||||
else:
|
||||
total_time += number
|
||||
|
||||
logging.info(f'Timer required for {total_time} seconds')
|
||||
|
@ -0,0 +1,15 @@
|
||||
{
|
||||
"scriptFile": "__init__.py",
|
||||
"bindings": [
|
||||
{
|
||||
"type": "eventHubTrigger",
|
||||
"name": "events",
|
||||
"direction": "in",
|
||||
"eventHubName": "samples-workitems",
|
||||
"connection": "IOT_HUB_CONNECTION_STRING",
|
||||
"cardinality": "many",
|
||||
"consumerGroup": "$Default",
|
||||
"dataType": "binary"
|
||||
}
|
||||
]
|
||||
}
|
@ -0,0 +1,33 @@
|
||||
# Set a timer and provide spoken feedback
|
||||
|
||||
Add a sketchnote if possible/appropriate
|
||||
|
||||

|
||||
|
||||
## Pre-lecture quiz
|
||||
|
||||
[Pre-lecture quiz](https://brave-island-0b7c7f50f.azurestaticapps.net/quiz/33)
|
||||
|
||||
## Introduction
|
||||
|
||||
In this lesson you will learn about
|
||||
|
||||
In this lesson we'll cover:
|
||||
|
||||
* [Thing 1](#thing-1)
|
||||
|
||||
## Thing 1
|
||||
|
||||
---
|
||||
|
||||
## 🚀 Challenge
|
||||
|
||||
## Post-lecture quiz
|
||||
|
||||
[Post-lecture quiz](https://brave-island-0b7c7f50f.azurestaticapps.net/quiz/34)
|
||||
|
||||
## Review & Self Study
|
||||
|
||||
## Assignment
|
||||
|
||||
[](assignment.md)
|
@ -0,0 +1,9 @@
|
||||
#
|
||||
|
||||
## Instructions
|
||||
|
||||
## Rubric
|
||||
|
||||
| Criteria | Exemplary | Adequate | Needs Improvement |
|
||||
| -------- | --------- | -------- | ----------------- |
|
||||
| | | | |
|
@ -0,0 +1,33 @@
|
||||
# Support multiple languages
|
||||
|
||||
Add a sketchnote if possible/appropriate
|
||||
|
||||

|
||||
|
||||
## Pre-lecture quiz
|
||||
|
||||
[Pre-lecture quiz](https://brave-island-0b7c7f50f.azurestaticapps.net/quiz/33)
|
||||
|
||||
## Introduction
|
||||
|
||||
In this lesson you will learn about
|
||||
|
||||
In this lesson we'll cover:
|
||||
|
||||
* [Thing 1](#thing-1)
|
||||
|
||||
## Thing 1
|
||||
|
||||
---
|
||||
|
||||
## 🚀 Challenge
|
||||
|
||||
## Post-lecture quiz
|
||||
|
||||
[Post-lecture quiz](https://brave-island-0b7c7f50f.azurestaticapps.net/quiz/34)
|
||||
|
||||
## Review & Self Study
|
||||
|
||||
## Assignment
|
||||
|
||||
[](assignment.md)
|
@ -0,0 +1,9 @@
|
||||
#
|
||||
|
||||
## Instructions
|
||||
|
||||
## Rubric
|
||||
|
||||
| Criteria | Exemplary | Adequate | Needs Improvement |
|
||||
| -------- | --------- | -------- | ----------------- |
|
||||
| | | | |
|
Binary file not shown.
After Width: | Height: | Size: 21 KiB |
After Width: | Height: | Size: 48 KiB |
After Width: | Height: | Size: 176 KiB |
Before Width: | Height: | Size: 358 KiB |
After Width: | Height: | Size: 39 KiB |
Some files were not shown because too many files have changed in this diff Show More
Loading…
Reference in new issue